A deep understanding of deep neural networks (DNNs) aids in efficient AI development by optimizing resource usage like computing power, data, and time.
Knowing the ins and outs of these networks allows for accurate modeling and precision in AI results, which expedites development and improves overall productivity in the generative AI sector.
How does in-depth knowledge of DNNs streamline AI model creation?
Understanding the architecture and functioning of DNNs forms the foundation for efficient model creation. It contributes to a precise configuration of different layers and nodes in the network.
Your knowledge saves unnecessary trial-and-error, accelerates model design, and provides quick insights into the model’s performance. 💡📈
Further, understanding DNNs brings more predictability to model behavior, reducing uncertainties in AI development. You can devise modifications or upgrades more confidently and also preempt potential pitfalls, thereby improving the development rate.
How can thorough DNN knowledge optimize resource usage in AI development?
Comprehending DNNs helps to optimize resource usage, a critical factor in AI development. Detailed knowledge allows for improved allocation of computational resources, offering efficient solutions and avoiding wastages.
Additionally, with deep understanding, one can make better use of datasets 📊🧠. A more intelligent positioning of data in the network, coupled with an informed selection of trainable parameters, ensures streamlined development and enhanced productivity in generative AI systems.
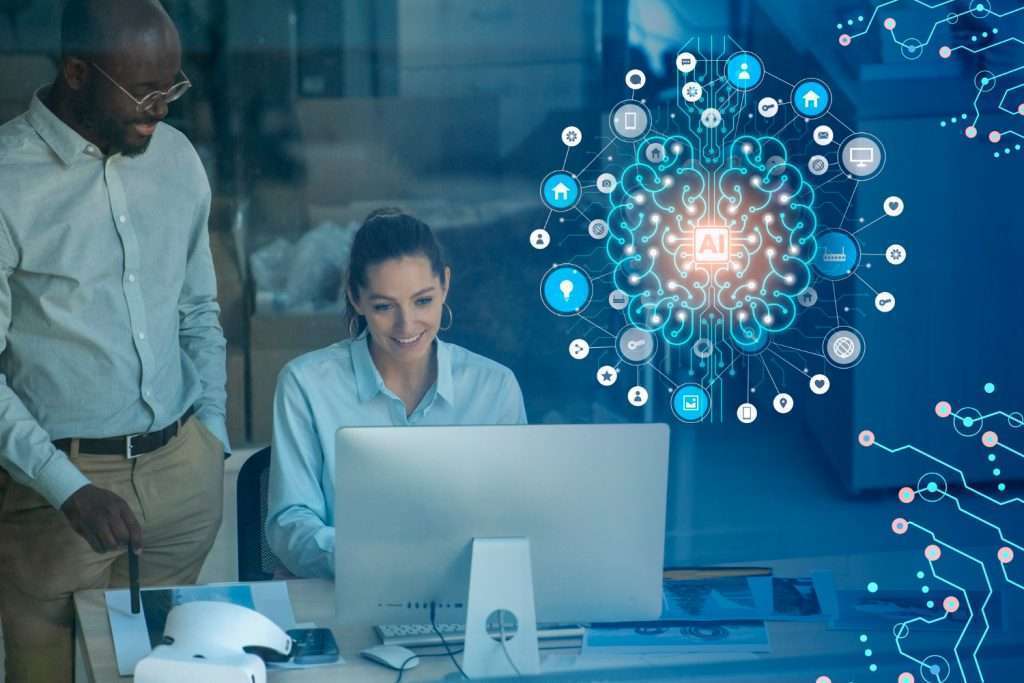
How does knowing well DNNs contribute to the accuracy and precision of AI outputs?
When you understand the DNNs, you can tune the various network parameters with more precision. This results in minimal error and deviation in AI outputs.
This is a direct consequence of the knowledge-accuracy correlation in any high-caliber AI-driven system, as detailed in our ‘Fundamental Elements of a Deep Neural Network in Generative AI‘ pillar article. 📚🔍
Moreover, knowing DNNs well contributes to consistent accuracy across different AI applications. It fosters a flexible framework for creating AI solutions that maintain high precision across different datasets or under varying environmental conditions.
How does proficiency in DNNs drive innovation in AI development?
Grasping the intricacies of DNNs can be a catalyst for innovation in AI development. It allows developers to conjure unconventional configurations and novel algorithmic structures that could drive the next breakthrough in generative AI. 💭🔍
Also, by understanding the basics, developers can adapt their models to suit new data types and contexts, opening up new possibilities in AI applications.
As per Andrew Ng, a prominent AI leader, “having a good intuition of how neural networks work is tremendously useful in designing and training these models.”
Conclusion
A sound knowledge of deep neural networks paves the way to efficiencies in AI development – from resource optimization to innovative solutions.
Understanding the structure and functioning of these networks enables precise model designs, quick insights into the model’s performance, and better resource management.
Furthermore, it elevates the accuracy and consistency of AI outcomes while inspiring innovation in AI applications. Knowledge is indeed power when it comes to honing efficiency in AI development. 🎯🚀
- Blockchain-Enabled IoT Device Authentication - October 16, 2024
- Quantum Cryptography: The Future of Secure Communication - October 9, 2024
- Photon Mapping for Enhanced Ray Tracing - October 2, 2024