Introduction to Deep Learning
Imagine a technological advancement that can simulate human learning processes. A technology that can transform simple inputs into more complex and composite outputs. This isn’t science fiction; this is the reality of a Deep Neural Network in Generative AI.
When considering the most significant developments in technology, one cannot ignore the rapid advancements and potential of deep learning. Drawn from the realms of Artificial Intelligence (AI), deep learning embodies the next technological frontier. Simulating human learning, this facet of AI offers real-world solutions for a multitude of tasks and challenges.
Deep learning’s foundation is based on Artificial Neural Networks. Paired with representation learning, these networks build upon the power of machines in software or hardware to simulate basic human learning processes. But what precisely makes these networks ‘deep’? The answer to this is simple – it’s the number of layers in the network.
Building Blocks of a Deep Neural Network
A deep neural network is a network with multiple layers – these can range from a few to several thousand. Signals pass through these layers from the input (first layer) through to the output. Data may traverse these layers multiple times, depending upon the specific function of the network.
The presence of numerous layers aids in the composition of features from lower layers. The data flows from the input layer to the output layer without looping back, this is known as a feedforward network.
However, deep neural networks are not mere constructs of multiple layers. They embody a complex system of interconnected artificial neurons, mirroring biological neurons present in the brain. These networks encompass millions of connections, although this figure is still orders of magnitude less than human-brained neurons.
Scope of Deep Learning in the Generative AI Industry
In the world of AI, deep learning has significant potential. For instance, these networks can identify faces, recognize speech, aid in machine translation, and support other complex tasks that humans find challenging.
Furthermore, Generative Adversarial Networks (GANs), a form of AI developed by Ian Goodfellow and his colleagues, embody an adversarial neural network contesting with each other in the form of a zero-sum game.
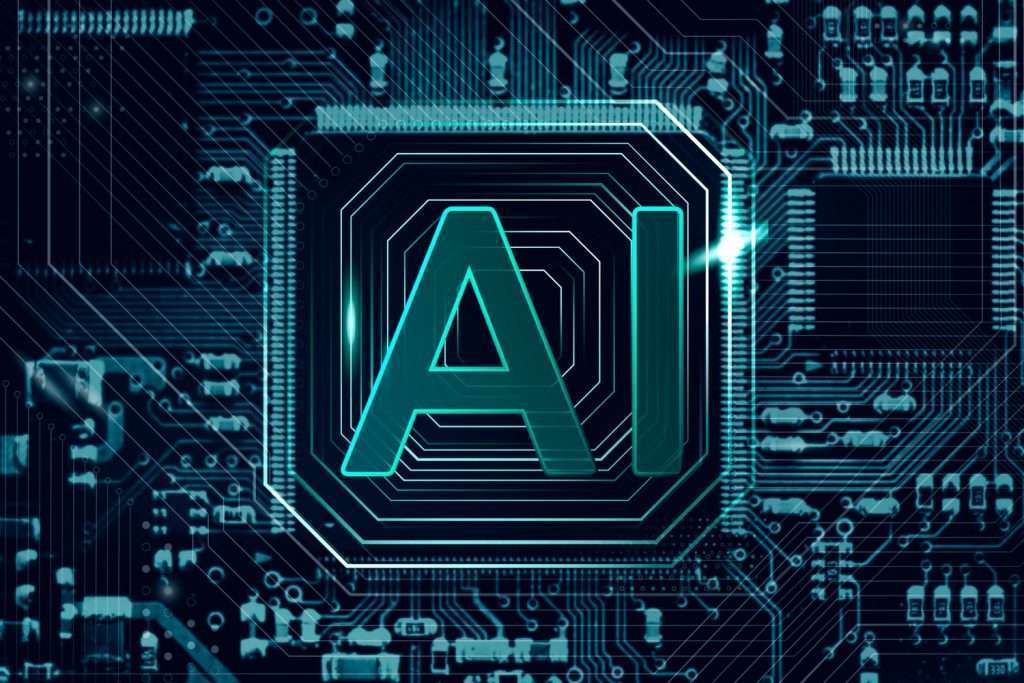
Deep neural networks have also demonstrated unprecedented results in large-scale image and speech recognition competitions. Networks like DanNet and AlexNet have consistently surpassed conventional methods in these contests. Such applications attest to the transformative impact that deep neural networks generate when applied to real-world challenges.
Navigating the Complexities: Unveiling the Power of Deep Neural Networks
The design and functioning of a deep neural network may appear incredibly complex and daunting. However, it’s essential to remember that each layer undertakes a simple mathematical manipulation. There are many training parameters to consider, such as the layer sizes, learning rate, and initial weights.
Building a deep neural network involves arranging layers into a hierarchy, streamlining CAP depth (the chain of transformations from input to output), and optimizing feature placement to various levels.
Certain aspects of these networks still require manual tuning, such as determining the number of layers or layer sizes that can provide varying degrees of abstraction.
Generative AI’s Future and Deep Neural Networks
From predicting diseases to designing robust marketing strategies, deep learning’s potential continues to grow. Industry data indicates that the impact of deep learning began around the early 2000s. Since then, deep neural nets now process approximately 10-20% of all checks written in the US.
As natural language processing and computer vision technologies evolve and mature, we anticipate even more applications for this promising technology.
In conclusion, the world of Generative AI and deep learning holds immense promise, promising to transform industries and the way we live and work. Despite the complexities, the fundamental elements of a deep neural network are quite straightforward.
With layers of interconnected neurons simulating human learning processes, deep neural networks offer a compelling blueprint of the future, today.
- Analyzing Patterns in Failed Products - July 25, 2024
- Hybrid Cryptographic Systems - July 24, 2024
- Inadequate Threat Intelligence Integration - July 23, 2024