If you’ve recently delved into the dynamic sphere of tech innovations, you’re likely to have encountered the question “What is deep learning?” This isn’t just a fleeting trend but is rapidly becoming the cornerstone of modern AI and machine learning.
Deep learning, which involves the use of neural networks to simulate human-like decision-making processes, promises to revolutionize how machines comprehend and interact with the world.
The Essence of Deep Learning
Building the Neural Foundations
At its core, deep learning is a subset of machine learning inspired by the human brain’s neural networks. This enables machines to make remarkably accurate predictions and decisions.
By emulating layers of neural networks, deep learning systems self-learn from data patterns, becoming increasingly proficient over time.
Constructing a neural network involves layers discerning various data aspects. Neural networks, serving as workhorses for deep learning, excel in tasks like recognizing voice commands in digital assistants and precisely detecting fraudulent activities.
Advancements in Deep Learning Technologies
Recent years have seen unprecedented advances in deep learning techniques. Highly sophisticated neural network architectures, such as Convolutional and Recurrent Neural Networks (CNNs and RNNs), have offered nuanced solutions to complex problems.
For instance, CNNs, with their unparalleled adeptness in processing visual data, has become an instrumental technology in computer vision, driving progress in self-driving vehicles, and facial recognition systems.
Deep Learning’s Transformational Impact
Revitalizing Industries with AI
Deploying deep learning techniques has wide-ranging implications across sectors. In healthcare 👨⚕, AI-driven models aid radiologists in swiftly detecting diseases from imaging data, potentially saving lives.
Furthermore, in finance 📈, deep learning’s predictive analytics reshape pathways for algorithmic trading and risk management, showcasing its transformative influence.
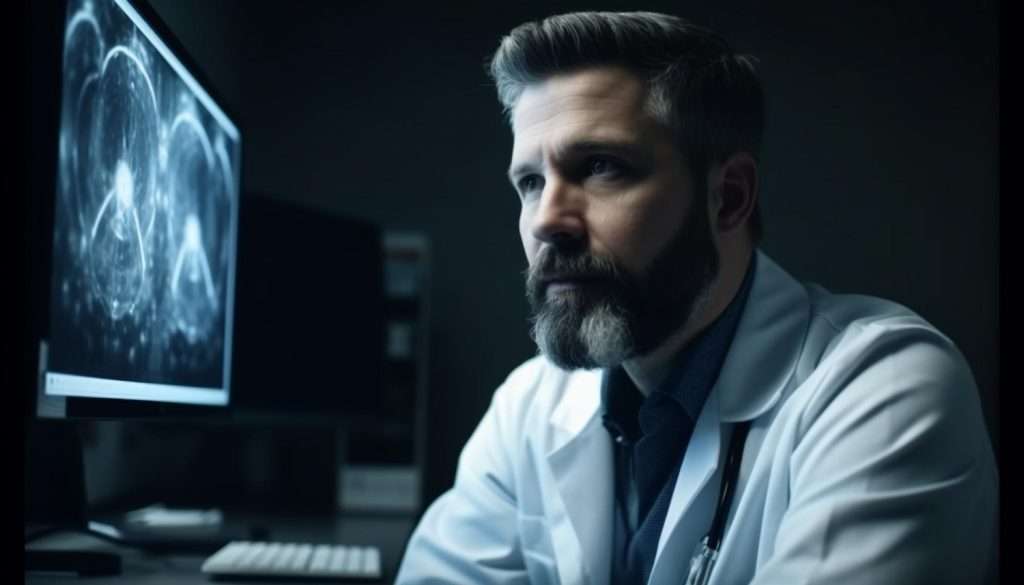
Creative Pursuits and Personalization
Moreover, the creative industries have not remained untouched. Deep learning algorithms extend creativity, refining content creation. They personalize movie recommendations and transform digital artwork using neural style transfer techniques. 🎬🎨
Customer experiences are sophisticatedly tailored as deep learning-powered chatbots interpret queries almost human-like. These techniques signify a personalized approach to interactions.
Implementing and Optimizing Techniques
Strategies for Refined Accuracy
To optimize deep learning models, several strategies are implemented, ranging from data pre-processing to the artful orchestration of training datasets. The models undergo regularization and hyperparameter tuning to achieve precision and efficiency in their problem-solving prowess.
Moreover, Frameworks such as TensorFlow provide the necessary tools for tech professionals to refine their models diligently, ensuring the extracted predictions stand up to real-world applicability and scrutiny.
Tackling Challenges with Innovation
Despite the strides made, the growth trajectory of deep learning isn’t devoid of challenges.
The requirement for vast datasets and substantial processing power continues to be a hurdle that needs continuous innovation and resource optimization.
Yet, with every obstacle confronted, deep learning experts innovate—introducing newer architectures and learning paradigms that extend the boundaries of what machines can achieve through iterative learning.
Applications and Real-world Examples
Customer Interactions Reimagined
In the customer service spectrum, deep learning breathes new life into interactions. The retail giant Amazon utilizes deep learning algorithms in Alexa to enhance user interaction through natural language processing, setting a new standard in customer engagement.
Such applications underline the undeniable pertinence of deep learning in sculpting not only business strategies but also in redefining user experiences in ways previously imagined only in the realm of science fiction.
Automotive Safety and Navigation
In autonomous driving, companies like Tesla use deep learning to interpret sensory data, enabling vehicles to navigate with futuristic acumen.
The fusion of sensors and deep learning is a safety and innovation beacon in the automotive industry. It illustrates how applying these models extensively impacts consumers and society.
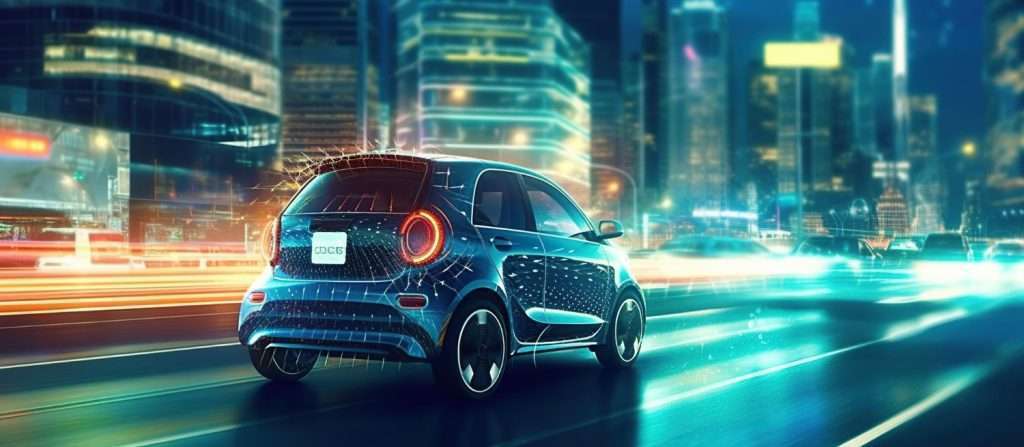
Conclusion: The Confluence of Learning and Innovation
Deep learning has undeniably carved a niche in the landscape of AI, offering robust solutions to intricate problems that once eluded the grasp of traditional computing paradigms.
As we’ve explored, the journey through neural networks, optimization techniques, industry applications, and real-world implications has highlighted the profound relevance of what is deep learning in today’s tech-dominated world.
Indeed, the nexus of deep learning with various domains from healthcare to automotive safety is a testament to its pivotal role in driving innovation and enhancing human-machine interaction.
For the seekers of knowledge within the tech ecosystem, unlocking the intricacies of deep learning equips them with a transformative toolkit that promises to redefine the frontiers of technology and beyond.
- Blockchain-Enabled IoT Device Authentication - October 16, 2024
- Quantum Cryptography: The Future of Secure Communication - October 9, 2024
- Photon Mapping for Enhanced Ray Tracing - October 2, 2024