GPT or LLM—these acronyms are at the forefront of AI research, especially with OpenAI’s groundbreaking advancements. Understanding the nuances between Generative Pre-trained Transformers (GPT) and Large Language Models (LLM) is crucial for anyone navigating the AI landscape.
Understanding GPT and LLM
OpenAI developed Generative Pre-trained Transformers (GPT) as a specific type of Large Language Model (LLM). In other words, GPT models, like GPT-3, generate human-like text based on what they’re given. They leverage the Transformer architecture, which excels at handling sequences of data, making them powerful tools for natural language processing (NLP) tasks.
However, Large Language Models (LLMs) encompass a wider range of models, including GPT. Designed for scalability and versatility, LLMs go beyond text generation. They can perform tasks like sentiment analysis, text summarization, and language translation. To learn more about how LLMs work, check out GrowthLoop’s comprehensive guide.
Both GPT and LLMs offer unique strengths and applications. Understanding the distinction between them is crucial to maximizing their potential.
Key Differences Between GPT and LLM
Here are some critical distinctions between GPT and LLM:
- Training Data and Scale: GPT-3, a massive LLM, devours 570GB of text data for pre-training (internet text, books, articles). LLMs, however, can vary in scale and training data, ranging from smaller models to extremely large ones. See Olga Green’s comparison for details.
- Architecture and Functionality: GPT models focus on the Transformer architecture for NLP tasks. LLMs are more flexible, using Transformers, RNNs, and CNNs for various goals. This allows them to be fine-tuned beyond just generating text.
- Use Cases and Applications: GPT models shine in text generation, making them useful for content creation, chatbots, and translation. LLMs, as more general tools, tackle a broader range of NLP tasks like sentiment analysis and text classification. See Amara’s article for a deeper dive.
- Ethical and Societal Implications: Both GPT and LLMs raise ethical concerns related to biases, misinformation, and misuse. Therefore, the responsible use of these models is crucial, especially in content creation and other sensitive applications.
Case Study: OpenAI’s GPT-4
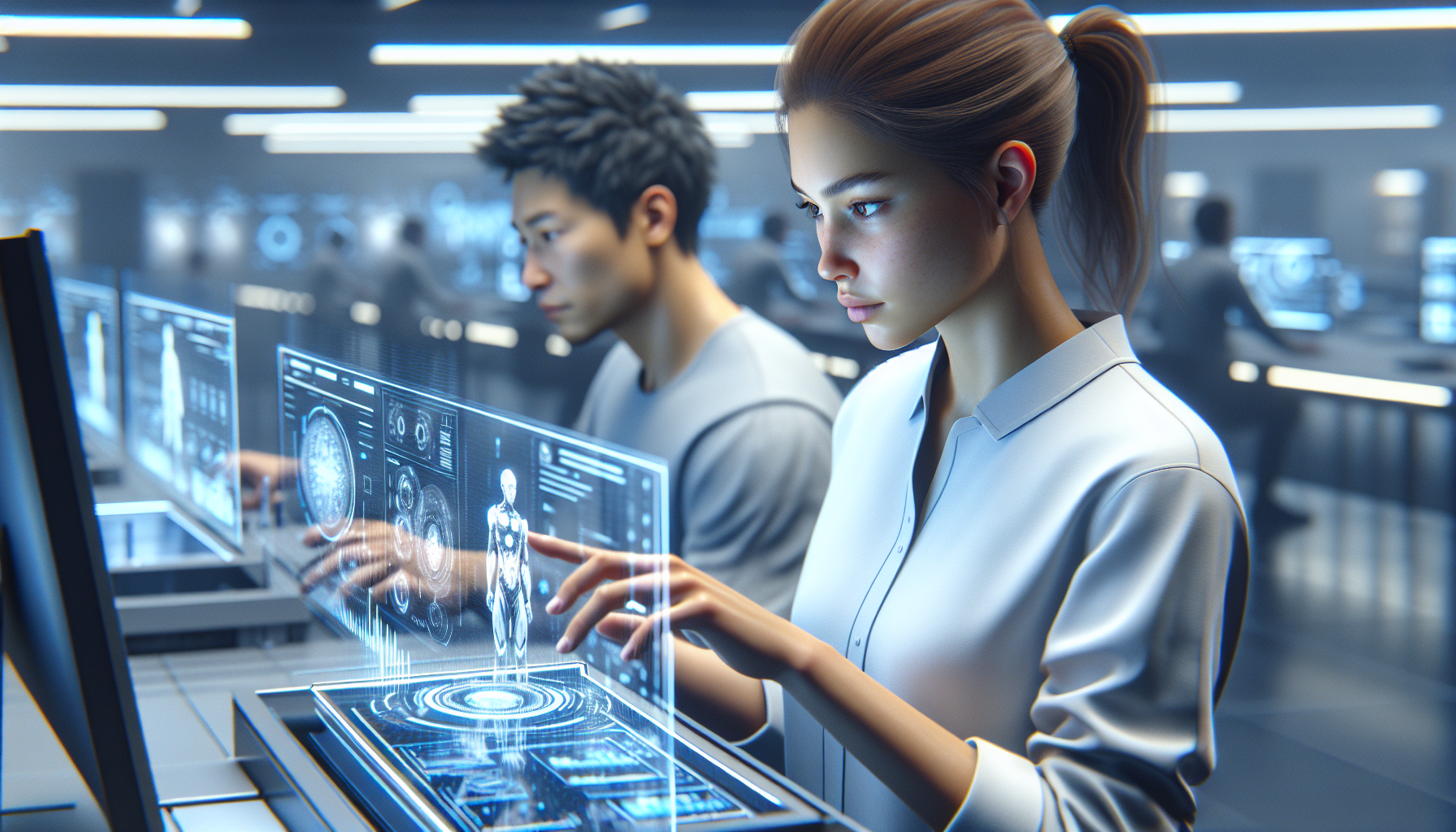
While OpenAI’s GPT-3 was a groundbreaking achievement, the landscape of large language models has evolved. In March 2023, OpenAI released GPT-4, its successor boasting even greater capabilities.
Building on GPT-3’s foundation, GPT-4 offers significant advancements. It excels at creative tasks, collaborating with users on projects like songwriting or screenplay writing. Additionally, GPT-4 handles much larger amounts of text, enabling long-form content creation, extended conversations, and in-depth document analysis.
Similar to GPT-3, GPT-4 has a wide range of applications. However, its enhanced abilities open doors to even more possibilities. Despite its potential, concerns around bias and misuse remain. OpenAI actively addresses these issues through safety measures and responsible use guidelines. The development of GPT-4 exemplifies the ongoing progress and challenges in deploying powerful language models for real-world use.
Future Predictions for GPT and LLM
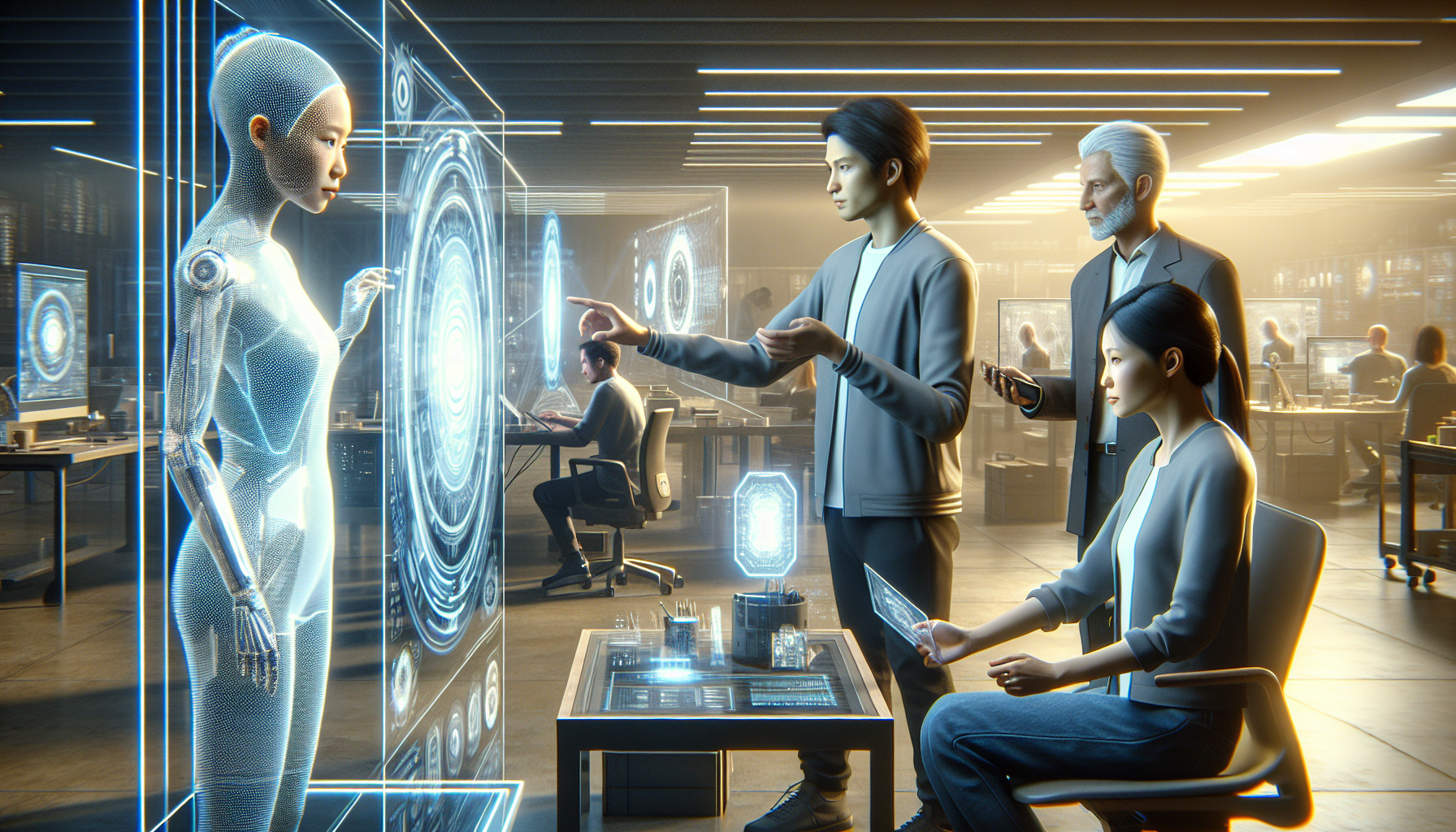
The future of GPT and LLM is promising, with several trends and developments to watch for:
- Increased Model Size and Capabilities: Expect GPT and LLMs to grow in size and capability, leading to more advanced applications.
- Enhanced Ethical Guidelines: With increased use, focus on responsible development and ethical guidelines will be paramount.
- Integration with Other Technologies: Integration with other technologies like robotics will create powerful AI systems.
- Domain-Specific Models: Domain-specific models tailored to healthcare, finance, etc. will become the norm.
- Improved User Interfaces: Easier interaction with GPT and LLMs will open them to a wider range of users.
Conclusion
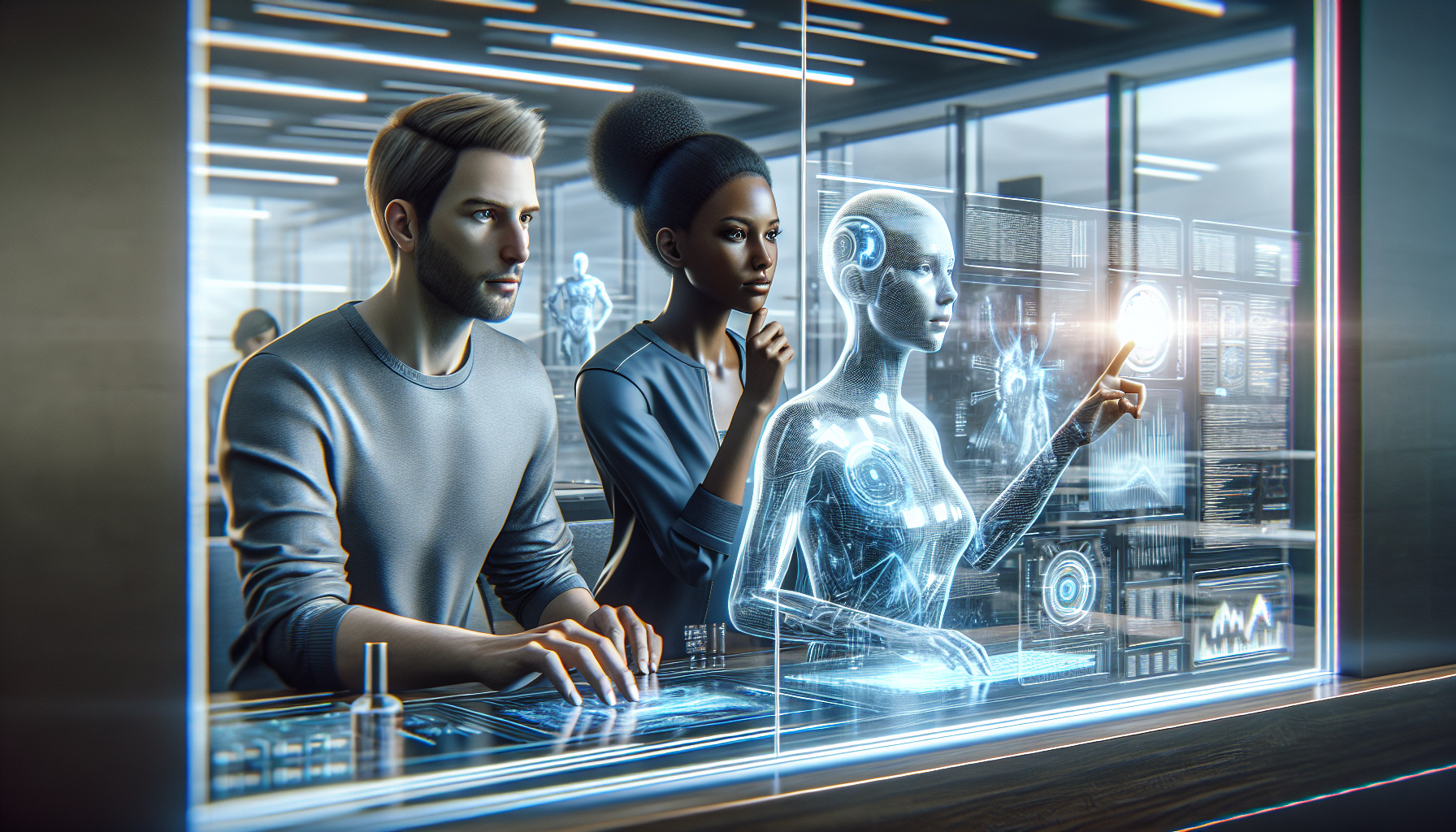
Understanding the differences between GPT and LLM is essential for leveraging their full potential. While GPT models, like GPT-3, are celebrated for their text-generation capabilities, LLMs offer a broader range of applications. As AI research continues to advance, we can expect even more sophisticated models and applications, along with a stronger emphasis on ethical guidelines and responsible usage.
For those in the AI content generation industry, staying informed about these developments is crucial. By understanding the capabilities and limitations of GPT and LLM, you can make more informed decisions about their use in your projects and applications.
References
- Understanding the Difference Between GPT and LLM: A Comprehensive Comparison | by Olga Green | Medium – A detailed comparison of GPT and LLM, highlighting their differences and applications.
- Large language model (LLM): How does it work? | GrowthLoop – An in-depth guide on how LLMs work and their various applications.
- What is the difference between GPT vs LLM? | by Amara | Medium – An article exploring the distinctions between GPT and LLM.
- Large language models (LLMs) vs. ChatGPT | ThoughtSpot – A comparison of LLMs and ChatGPT, discussing their unique features and applications.
- Large language model – Wikipedia – A comprehensive overview of large language models, their history, and their impact on AI research.
- Blockchain-Enabled IoT Device Authentication - October 16, 2024
- Quantum Cryptography: The Future of Secure Communication - October 9, 2024
- Photon Mapping for Enhanced Ray Tracing - October 2, 2024