Yes, there are various methods for detecting deepfakes.
As generative AI continues to advance, the ability to create realistic synthetic media known as deepfakes has greatly improved. Consequently, the tech industry is growing increasingly interested in developing and implementing techniques for identifying these deceptive creations.
This interest is especially pertinent for startups and medium enterprises in tech hubs, where innovation and ethical use of AI are critical for business integrity and consumer trust.
How do machine learning algorithms identify deepfakes?
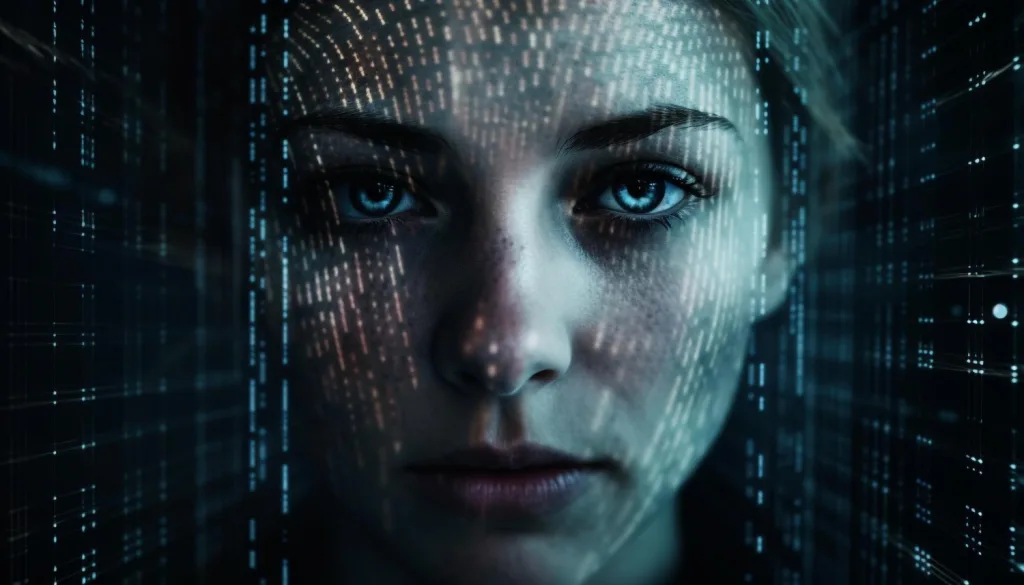
Machine learning algorithms can identify deepfakes by analyzing patterns and inconsistencies that are difficult for the human eye to detect.
By training on datasets of real and fake content, these algorithms learn to spot subtle differences in facial expressions, head movements, and even blinking patterns that are not typical in natural human behavior.
Moreover, they can examine pixel-level details and the quality of images or videos to discover traces left by deepfake generation processes.
For example, some deepfake detection systems use convolutional neural networks (CNNs) to distinguish between authentic and manipulated content.
These networks may focus on artifacts introduced during the image generation process, such as inconsistent lighting, unnatural skin tones, or warped backgrounds. These signs, while often imperceptible to viewers, can be strong indicators to the trained models that content could be artificial.
What are the challenges in detecting deepfakes?
Despite the progress made in detection technology, deepfakes continue to pose a significant challenge. A primary issue is the ever-improving quality of deepfake generators, leading to a cat-and-mouse game between creators and detectors.
The deepfakes are becoming increasingly sophisticated, mimicking human characteristics more accurately and therefore becoming harder to differentiate from authentic media.
Additionally, the variability in video quality, different compression rates, and diverse facial angles or lighting conditions can impact the performance of deepfake detection tools.
Adversarial methods, where deepfake creators use similar machine learning techniques to evade detection, also present a formidable challenge. These methods can alter deepfakes to avoid recognized patterns picked up by detection systems, compelling developers to continually update and refine their detection algorithms.
Can blockchain technology help verify the authenticity of media?
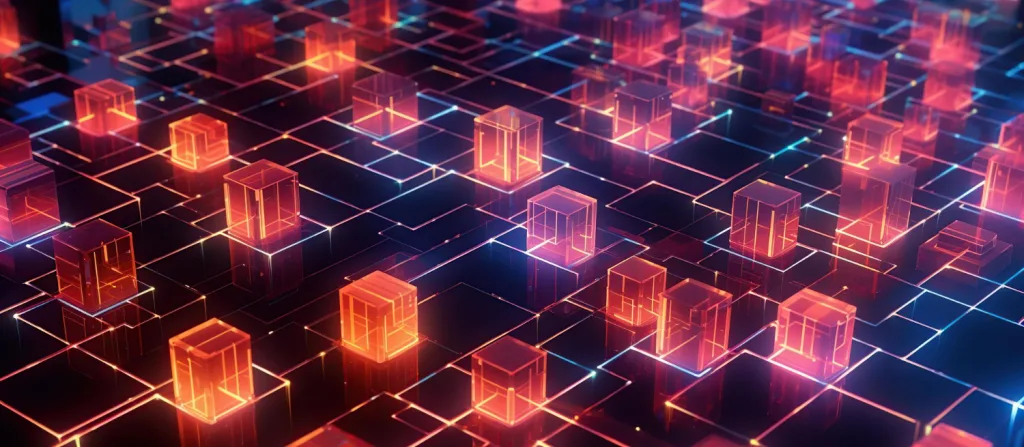
Blockchain technology is gaining attention as a potential solution to verify the authenticity of digital media.
By creating an immutable ledger of content, blockchain can help to establish a chain of custody for a digital asset. This means that every time a piece of media is created, edited, or shared, it can be recorded on the blockchain, ensuring traceability and transparency.
When combined with cryptographic signatures, this technology can enable content creators to certify their work, and consumers can check the blockchain to verify its origin and history.
Although blockchain does not detect deepfakes in the conventional sense, it helps authenticate genuine content and could serve as a preventative measure against the spread of deepfakes by enabling a verifiable source of truth.
What future developments are expected in deepfake detection?
Looking ahead, we can expect significant advancements in deepfake detection as researchers and tech companies invest in more sophisticated techniques.
Improvements in AI, enhanced training datasets, and cross-industry collaborations will likely contribute to the development of more accurate and efficient detection systems. 🤖
Additionally, as deepfakes become more common, public awareness and education will play a crucial role in mitigating the spread of deceptive content.
One promising area of development is the use of biometric analysis, which can detect the unique biological responses and traits that deepfakes cannot perfectly replicate.
There’s also ongoing work in developing standardized benchmarks for deepfake detection, which would help in evaluating and improving the robustness of detection tools against various deepfake methods.
As these technologies evolve, it’s clear that the arms race between deepfake creation and detection will continue to inspire innovation in the field. 🔍💻
Conclusion
While deepfakes present a formidable challenge in the digital world, various methods are currently available and in development to detect and mitigate their spread. These methods include machine learning algorithms, adversarial models, and potential blockchain verification systems.
The continued evolution of deepfake technology demands an ongoing commitment to improving detection methods. 🚀
Awareness, education, and research are key components in keeping ahead of this issue, ensuring integrity and trust in digital media. For a closer look at the implications of generative AI and deepfakes across the tech industry, visit our pillar articles on deepfake examples in generative AI.
- Blockchain-Enabled IoT Device Authentication - October 16, 2024
- Quantum Cryptography: The Future of Secure Communication - October 9, 2024
- Photon Mapping for Enhanced Ray Tracing - October 2, 2024