AI bias detection is crucial for ensuring fairness and accuracy in automated decision-making processes. This article explores the importance of identifying and mitigating biases in AI systems, providing insights and practical steps for professionals in the machine learning and artificial intelligence industry.
Understanding AI Bias Detection and Mitigation
Key Points
- Bias in AI can lead to unfair and discriminatory outcomes.
- Historical data and incomplete training sets are common sources of bias.
- Regular audits and diverse teams can help identify and mitigate bias.
- Ethical frameworks are essential for guiding AI development.
- Proactive measures can prevent bias from affecting AI decisions.
Definition and Importance
AI bias detection refers to the process of identifying and addressing biases in artificial intelligence systems. Biases can arise from various sources, including historical data, incomplete training sets, and the inherent prejudices of the developers. Detecting these biases is crucial because they can lead to unfair and discriminatory outcomes, affecting individuals and groups negatively.
Bias in AI systems can manifest in different ways, such as gender bias in hiring algorithms or racial bias in facial recognition software. These biases can perpetuate existing inequalities and create new forms of discrimination. Therefore, it is essential to implement robust bias detection mechanisms to ensure that AI systems are fair and equitable.
Mitigating bias in AI involves not only detecting it but also taking proactive measures to address and prevent it. This includes using diverse training data, implementing regular audits, and adhering to ethical guidelines. By doing so, AI developers can create systems that are more accurate, reliable, and fair.
Historical Context
Historically, biases in AI systems have often gone unnoticed until they caused significant harm. For example, the COMPAS algorithm used in the criminal justice system was found to be biased against African Americans, leading to unfair sentencing. Similarly, facial recognition technologies have been criticized for their inability to accurately identify individuals with darker skin tones.
These historical examples highlight the importance of proactive bias detection and mitigation. By learning from past mistakes, AI developers can implement better practices and create more equitable systems. This involves not only technical solutions but also a commitment to ethical principles and social responsibility.
In recent years, there has been a growing awareness of the need for bias detection in AI. Organizations and researchers are developing new tools and methodologies to identify and address biases in AI systems. This ongoing effort is crucial for ensuring that AI technologies benefit all individuals and communities fairly.
Current Practices
Today, various techniques and tools are available for detecting and mitigating bias in AI systems. These include statistical methods, fairness metrics, and bias detection algorithms. For example, IBM’s AI Fairness 360 toolkit provides a comprehensive set of tools for assessing and mitigating bias in machine learning models.
Another important practice is the use of diverse and representative training data. By ensuring that training data includes a wide range of perspectives and experiences, developers can reduce the risk of bias in AI systems. Additionally, regular audits and evaluations can help identify and address biases that may arise during the development and deployment of AI technologies.
Ethical frameworks and guidelines also play a crucial role in bias detection and mitigation. Organizations like the European Union and the OECD have developed ethical guidelines for AI, emphasizing the importance of fairness, transparency, and accountability. By adhering to these guidelines, AI developers can create systems that are more equitable and just.
Challenges in AI Bias Detection
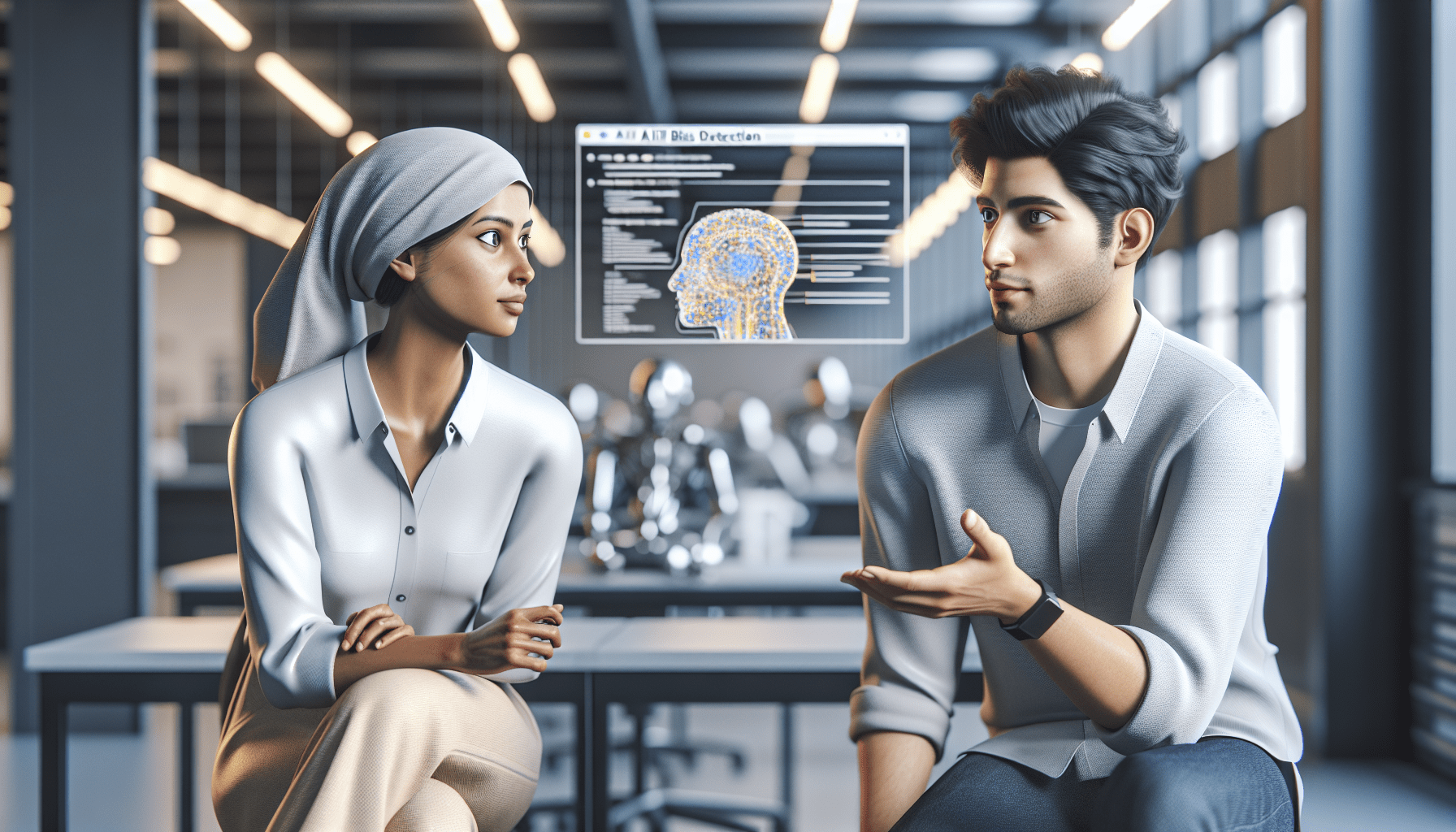
Data Quality and Representation
One of the most significant challenges in AI bias detection is ensuring the quality and representation of training data. Incomplete or unrepresentative data can lead to biased outcomes, as the AI system may not accurately reflect the diversity of the real world. For example, if a facial recognition system is trained primarily on images of light-skinned individuals, it may struggle to accurately identify individuals with darker skin tones.
To address this challenge, it is essential to use diverse and representative training data. This involves collecting data from various sources and ensuring that it includes a wide range of perspectives and experiences. Additionally, regular audits and evaluations can help identify and address biases that may arise from data quality and representation issues.
Algorithmic Transparency
Another challenge in AI bias detection is ensuring algorithmic transparency. Many AI systems operate as “black boxes,” making it difficult to understand how they make decisions. This lack of transparency can hinder efforts to detect and mitigate bias, as it is challenging to identify the sources of bias and take corrective actions.
To improve algorithmic transparency, developers can use techniques such as explainable AI (XAI) and model interpretability. These techniques provide insights into how AI systems make decisions, allowing developers to identify and address biases more effectively. Additionally, transparency can build trust with users and stakeholders, as they can better understand and evaluate the fairness of AI systems.
Ethical Considerations
Ethical considerations are also a significant challenge in AI bias detection. Ensuring fairness and equity in AI systems requires a commitment to ethical principles and social responsibility. This involves not only technical solutions but also a broader understanding of the social and cultural contexts in which AI systems operate.
Developers must consider the potential impacts of AI systems on different groups and communities, particularly those that are historically marginalized or disadvantaged. By adopting ethical guidelines and frameworks, developers can create AI systems that are more equitable and just. Additionally, engaging with diverse stakeholders and communities can provide valuable insights and perspectives, helping to identify and address biases more effectively.
Steps to Mitigate AI Bias
Step 1: Diverse Training Data
The first step in mitigating AI bias detection is to use diverse and representative training data. This involves collecting data from various sources and ensuring that it includes a wide range of perspectives and experiences. By doing so, developers can reduce the risk of bias in AI systems and create more accurate and fair models.
Additionally, regular audits and evaluations can help identify and address biases that may arise from data quality and representation issues. By continuously monitoring and updating training data, developers can ensure that AI systems remain fair and equitable over time.
Step 2: Algorithmic Transparency
Improving algorithmic transparency is another crucial step in mitigating AI bias detection. Techniques such as explainable AI (XAI) and model interpretability can provide insights into how AI systems make decisions, allowing developers to identify and address biases more effectively.
By making AI systems more transparent, developers can build trust with users and stakeholders, as they can better understand and evaluate the fairness of AI systems. Additionally, transparency can help identify and address biases that may arise during the development and deployment of AI technologies.
Step 3: Ethical Guidelines
Adopting ethical guidelines and frameworks is essential for mitigating AI bias detection. Organizations like the European Union and the OECD have developed ethical guidelines for AI, emphasizing the importance of fairness, transparency, and accountability.
By adhering to these guidelines, developers can create AI systems that are more equitable and just. Additionally, engaging with diverse stakeholders and communities can provide valuable insights and perspectives, helping to identify and address biases more effectively.
FAQs
What is AI bias detection?
AI bias detection refers to the process of identifying and addressing biases in artificial intelligence systems. Biases can arise from various sources, including historical data, incomplete training sets, and the inherent prejudices of the developers.
Why is AI bias detection important?
AI bias detection is crucial because biases in AI systems can lead to unfair and discriminatory outcomes, affecting individuals and groups negatively. Detecting and mitigating these biases ensures that AI systems are fair and equitable.
How can AI bias be mitigated?
AI bias can be mitigated by using diverse and representative training data, improving algorithmic transparency, and adopting ethical guidelines and frameworks. Regular audits and evaluations can also help identify and address biases in AI systems.
What are some common sources of AI bias?
Common sources of AI bias include historical data, incomplete training sets, and the inherent prejudices of the developers. Biases can also arise from unrepresentative data and lack of algorithmic transparency.
Future of AI Bias Detection
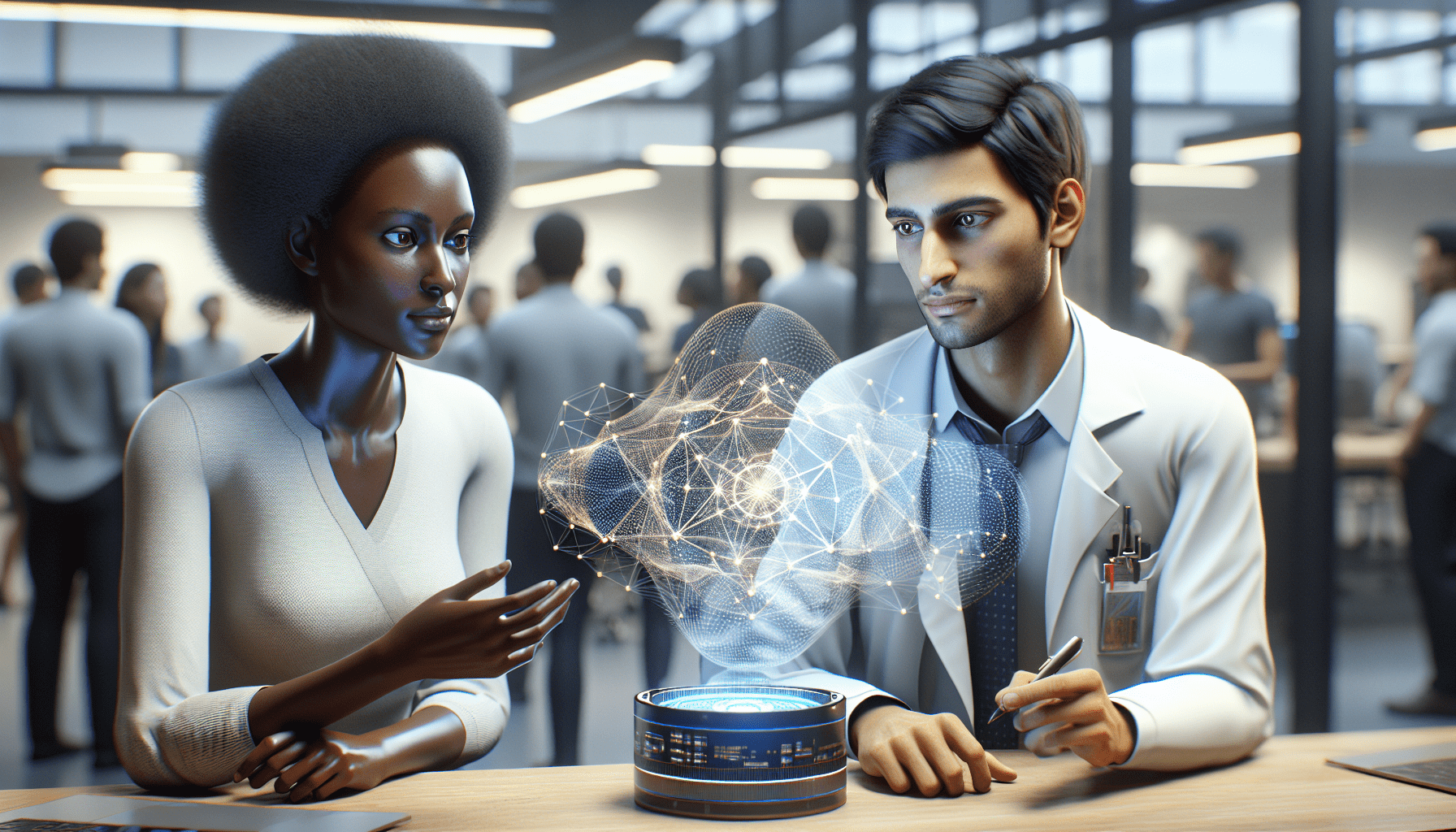
The future of AI bias detection is promising, with ongoing advancements in technology and methodologies. Here are five predictions for the future:
- Increased use of explainable AI (XAI): Techniques for making AI systems more transparent and understandable will become more widespread, helping to identify and address biases more effectively.
- Development of new fairness metrics: Researchers will continue to develop new metrics for assessing and mitigating bias in AI systems, leading to more accurate and fair models.
- Greater emphasis on ethical guidelines: Organizations and governments will place a greater emphasis on ethical guidelines and frameworks for AI, ensuring that AI systems are fair and equitable.
- Integration of bias detection tools: Bias detection tools will become more integrated into the AI development process, making it easier for developers to identify and address biases.
- Collaboration with diverse stakeholders: Engaging with diverse stakeholders and communities will become a standard practice, providing valuable insights and perspectives for identifying and addressing biases in AI systems.
More Information
- Algorithmic bias detection and mitigation: Best practices and policies to reduce consumer harms | Brookings – This article provides an in-depth analysis of best practices and policies for reducing consumer harms caused by algorithmic bias.
- AI-Detectors Biased Against Non-Native English Writers | Stanford HAI – This article discusses the biases in AI detectors against non-native English writers and the implications for fairness in AI systems.
- Bias Detection Tools in Health Care Challenge | National Center for Advancing Translational Sciences – This challenge encourages the development of bias-detection and correction tools for clinical decision support algorithms.
- Bias in AI: How to Mitigate Bias in AI Systems | Toptal® – This article provides practical strategies for mitigating bias in AI systems, with a focus on fairness and accuracy.
Disclaimer
This is an AI-generated article with educative purposes and doesn’t intend to give advice or recommend its implementation. The goal is to inspire readers to research and delve deeper into the topics covered in the article.
- Blockchain-Enabled IoT Device Authentication - October 16, 2024
- Quantum Cryptography: The Future of Secure Communication - October 9, 2024
- Photon Mapping for Enhanced Ray Tracing - October 2, 2024