The primary difference between Deep learning AI and traditional machine learning lies in their operational framework. Deep learning AI can autonomously identify patterns in large datasets. In contrast, traditional machine learning involves manual feature extraction and guided learning.
This distinction is critically important in security applications. The dynamic nature of threats requires systems that can learn and adapt without constant supervision.
How does deep learning AI enhance threat detection?
Deep learning AI transforms threat detection by significantly reducing false positives and identifying novel threats. Its ability to sift through massive amounts of data and learn from the information it processes enables it to recognize patterns and anomalies that would be imperceptible to traditional machine learning algorithms. This means it can adapt to new, previously unseen threats more quickly and with greater accuracy.
Moreover, deep learning AI systems continue to improve over time. As they encounter new threats, they adjust their models accordingly. This continuous learning loop ensures that the system becomes increasingly effective at identifying threats, vastly outpacing the capabilities of systems reliant on traditional machine learning methods.
What challenges does deep learning AI face in cybersecurity?
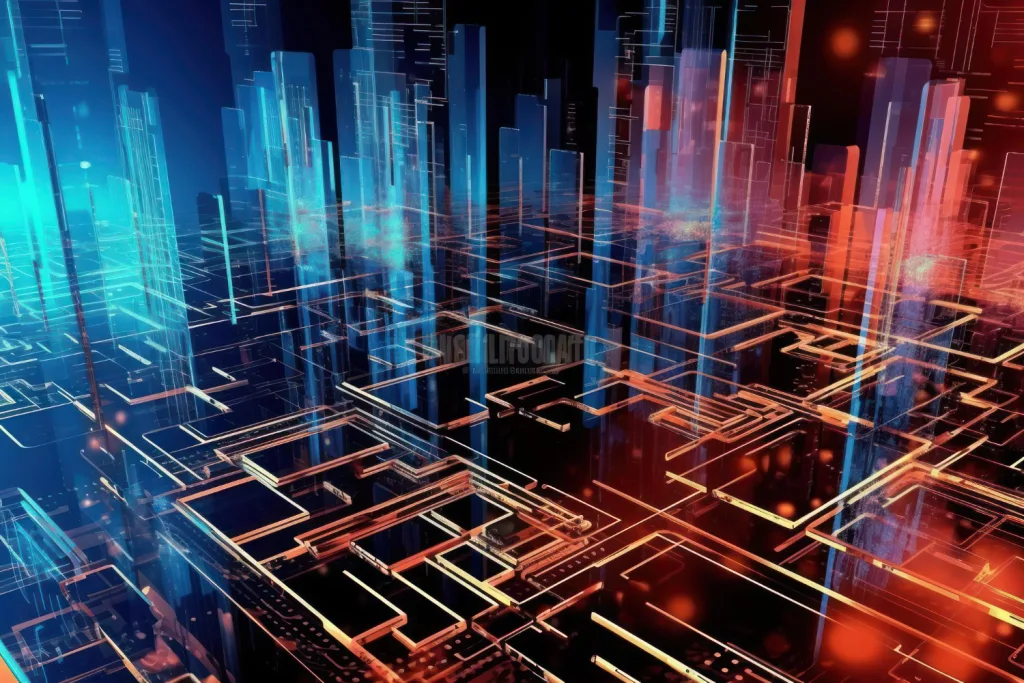
While deep learning AI offers substantial improvements over traditional methods, it’s not without its challenges. One of the primary concerns is the requirement for vast amounts of training data to effectively learn and make accurate predictions. In cybersecurity, acquiring large and representative datasets of malicious and benign activities can be difficult, limiting the initial effectiveness of deep learning systems.
Additionally, deep learning models are often described as “black boxes” because determining exactly how they make their decisions can be challenging. This opacity can be problematic in security settings where understanding the reasoning behind a threat detection is as crucial as the detection itself. Efforts to make these models more interpretable are ongoing, but it remains a notable challenge.
How does the adoption of deep learning AI affect incident response times?
One of the most significant benefits of implementing deep learning AI in security applications is the potential for drastically reduced incident response times. Deep learning models, with their ability to quickly sift through data and identify patterns, can detect threats at a pace that far exceeds human capabilities. This rapid detection allows for immediate remediation efforts, potentially stopping threats before they cause significant damage.
Additionally, because deep learning systems learn from each interaction, their ability to detect and respond to threats improves over time. This results in a more resilient security posture that can adapt to the ever-changing threat landscape faster than ever before, significantly improving overall security effectiveness.
What future advancements in deep learning AI are expected to impact security applications?
The future of deep learning AI in security applications looks promising. Several advancements are on the horizon. One significant advancement is the improvement of unsupervised learning algorithms. These algorithms do not require labeled datasets to learn. This improvement could alleviate the problem of acquiring vast amounts of labeled training data. As a result, deep learning AI will become even more effective in identifying novel threats.
Another anticipated advancement is the development of more transparent deep-learning models. As these models become more interpretable, their trust will likely increase within the cybersecurity community. This transparency will improve incident response strategies. Additionally, it will allow for a deeper understanding of the nature of threats, leading to more robust security measures.
Conclusion
Deep learning AI differs from traditional machine learning in its ability to autonomously identify patterns and learn from data. This capability is especially beneficial in security applications. It enhances threat detection, reduces incident response times, and adapts to new threats more efficiently.
However, challenges such as the need for large datasets and its “black box” nature persist. Advancements in unsupervised learning and model interpretability are expected to address these issues.
The continued evolution of deep learning AI promises to significantly impact cybersecurity strategies. IT managers, CTOs, and security analysts should focus on this critical area.
For a more detailed exploration into deep learning AI, consider reading Revolutionizing Tomorrow: Exploring the AI Frontier with Deep Learning.
- Burnout in Remote Teams: How It’s Draining Your Profits - January 27, 2025
- Signs You’re Understaffed - January 20, 2025
- The Cost of Silence: Communicating Negative Feedback - January 13, 2025