AI acts as a supercharger for edge computing in developing tech enterprises. Traditional cloud-based processing can be sluggish due to geographical distances and bandwidth limitations. AI on the edge solves this by analyzing data locally, right where it’s generated. This significantly reduces latency, allowing for real-time decision-making. Imagine a factory using AI to analyze sensor data from machines to predict equipment failure. With edge AI, the system can trigger immediate maintenance, preventing costly downtime.
Furthermore, AI is particularly beneficial for developing companies facing resource constraints. By processing data locally, edge AI reduces dependence on expensive cloud resources and bandwidth.
Additionally, AI algorithms can be trained on smaller datasets collected at the edge, a major advantage for companies that might not have the massive datasets needed for traditional AI models. This empowers developing tech enterprises to leverage the power of AI without needing huge upfront investments in infrastructure or data collection.
What specific AI tools are driving advancements in edge computing?
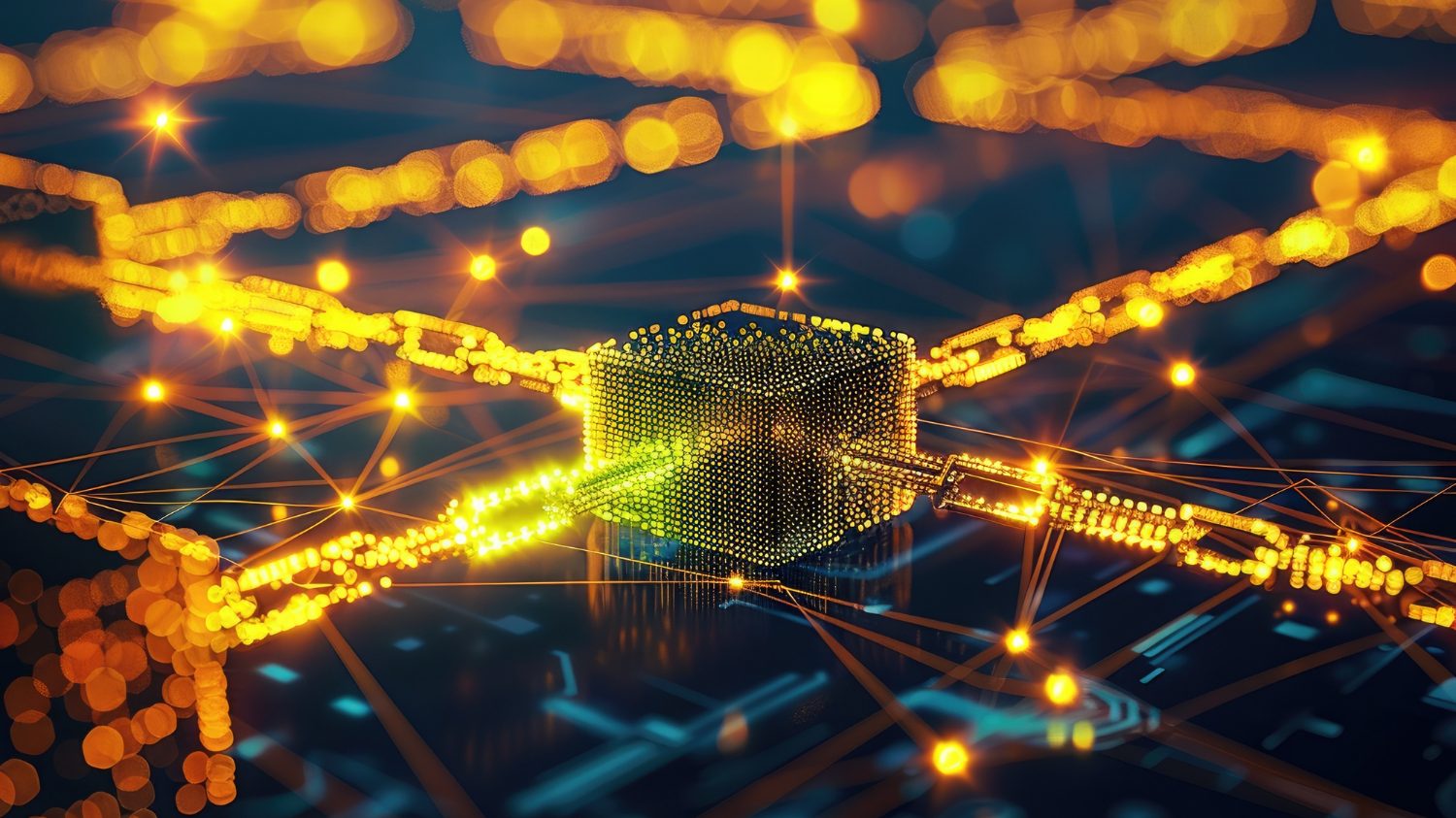
Machine learning algorithms and neural networks are core AI tools propelling edge computing forward. Machine learning algorithms optimize data processing and decision-making on edge devices by learning from data patterns, enabling these devices to perform tasks without sending data back to a central server for analysis.
Neural networks, particularly Convolutional Neural Networks (CNNs) for image and video data, allow edge devices to conduct complex processing locally. This capability is vital for applications like facial recognition and traffic flow analysis in smart cities.
Beyond core AI tools, advancements in model optimization techniques are crucial for edge computing. Techniques like pruning, quantization, and knowledge distillation reduce the size and complexity of AI models while maintaining accuracy. This allows even resource-constrained devices to run powerful AI applications.
Additionally, frameworks like TensorFlow Lite and ONNX Runtime are specifically designed for deploying AI models on edge devices, offering efficient execution and minimal resource footprint.
How can AI optimize energy consumption in edge computing for developing tech startups?
Developing tech startups are often conscious of their environmental impact and operational costs. AI can be a valuable tool for optimizing energy consumption in edge computing environments. Here are a couple of ways:
- Smart resource allocation: AI algorithms can analyze data on device workloads and power usage. Based on this data, AI can dynamically allocate resources to edge devices, ensuring optimal performance while minimizing energy consumption. For example, AI can power down underutilized devices or adjust processing power based on real-time needs.
- Predictive maintenance: As mentioned earlier, AI can be used for predictive maintenance of edge devices. By identifying potential issues before they occur, AI can help prevent unnecessary device failures and the associated energy waste. Additionally, AI can optimize maintenance schedules, reducing the number of service calls and overall energy consumption.
How does AI improve the security of edge computing environments?
AI brings several security benefits to edge computing environments, which typically handle data from numerous devices at the network’s periphery. By processing data locally on these devices, AI reduces the amount of sensitive information traveling across networks. This minimizes the risk of data breaches since hackers have fewer opportunities to intercept it.
Additionally, AI can analyze this data in real time, enabling it to detect anomalies and suspicious activity much faster. This allows for quicker intervention and helps prevent security incidents from escalating. For instance, AI can identify unusual patterns in sensor data or network traffic, potentially indicating a cyberattack in progress.
This is especially important for tech enterprises managing many devices connected to the Internet of Things (IoT). In these environments, the sheer number of devices can make traditional security measures cumbersome and slow. AI-powered edge computing offers a scalable and automated approach to securing these vast networks, ensuring the safety of sensitive data and the overall health of the system.
For a deeper understanding of the role of AI apps in tech company growth and finding the best solutions, head over to our pillar article: Top 5 AI Apps for Tech Companies to Drive Growth.
- Micro-Scale Biomimicry for Material Innovation - July 4, 2024
- Hyper-Personalized Content Creation Using User Behavioral Data - July 3, 2024
- Comparative Analysis of EMA and FDA Regulations in the Biotech Industry - June 28, 2024