Understanding Patient Data Privacy in AI Diagnostics
Key Points
- AI in healthcare requires large amounts of patient data to function effectively.
- Data privacy concerns are paramount when using AI for diagnostics.
- Regulations like GDPR play a crucial role in protecting patient data.
- Balancing data access and privacy is a significant challenge.
- Innovative solutions like generative data can help mitigate privacy risks.
Introduction to AI in Healthcare
AI is revolutionizing healthcare by providing advanced diagnostic tools that can analyze vast amounts of data quickly and accurately. These tools can assist in early detection of diseases, personalized treatment plans, and overall improved patient outcomes. However, the integration of AI in healthcare also brings significant challenges, particularly concerning patient data privacy.
AI systems require access to large datasets to train their algorithms effectively. This data often includes sensitive patient information, raising concerns about how this data is collected, stored, and used. Ensuring that patient data remains confidential and secure is a critical aspect of implementing AI in healthcare.
Regulations such as the General Data Protection Regulation (GDPR) in Europe and the Health Insurance Portability and Accountability Act (HIPAA) in the United States provide frameworks for protecting patient data. These regulations mandate strict guidelines on data handling, emphasizing the need for patient consent and data anonymization.
Importance of Data Privacy
Data privacy is a fundamental right that protects individuals from unauthorized access to their personal information. In the context of healthcare, data privacy is crucial because medical records contain highly sensitive information that, if misused, can lead to severe consequences for patients.
AI diagnostics rely on patient data to function, making it essential to implement robust data privacy measures. This includes ensuring that data is anonymized, encrypted, and stored securely. Additionally, patients should have control over their data, including the ability to consent to its use and withdraw consent if they choose.
Maintaining data privacy not only protects patients but also builds trust in AI technologies. When patients trust that their data is handled responsibly, they are more likely to consent to its use, enabling the development of more effective AI diagnostic tools.
Challenges in Ensuring Data Privacy
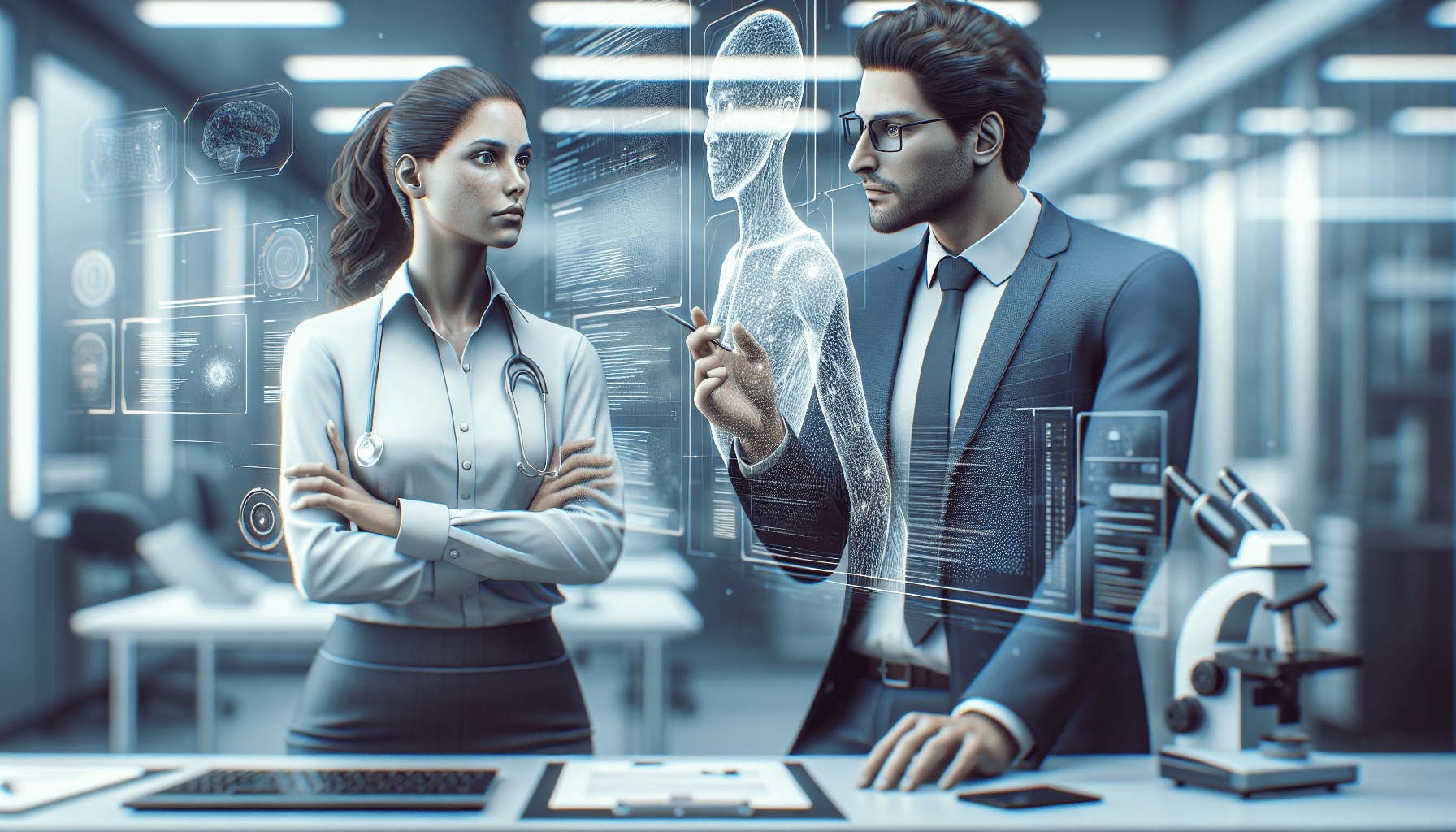
Data Breaches and Cybersecurity Threats
One of the most significant challenges in ensuring data privacy in AI diagnostics is the risk of data breaches and cybersecurity threats. Healthcare organizations are prime targets for cyberattacks due to the valuable information they hold. A data breach can lead to unauthorized access to patient records, resulting in identity theft, financial loss, and other severe consequences.
To mitigate these risks, healthcare organizations must invest in robust cybersecurity measures. This includes implementing advanced encryption techniques, regularly updating security protocols, and conducting thorough risk assessments. Additionally, staff should be trained on best practices for data security to prevent accidental breaches.
Regulatory Compliance
Compliance with data privacy regulations is another significant challenge. Regulations like GDPR and HIPAA set stringent requirements for data handling, and non-compliance can result in hefty fines and legal repercussions. Healthcare organizations must ensure that their AI systems comply with these regulations, which can be complex and resource-intensive.
Achieving compliance involves implementing comprehensive data protection policies, conducting regular audits, and staying updated on regulatory changes. Organizations may also need to work closely with legal experts to navigate the complexities of data privacy laws and ensure that their AI systems meet all necessary requirements.
Balancing Data Access and Privacy
AI diagnostics require access to large datasets to function effectively, but this need for data must be balanced with the need to protect patient privacy. Striking this balance is a significant challenge, as overly restrictive data access policies can hinder the development of AI technologies, while lax policies can compromise patient privacy.
Innovative solutions, such as the use of generative data, can help address this challenge. Generative models create synthetic data that mimics real patient data without containing any actual patient information. This allows AI systems to train on large datasets without compromising patient privacy. However, developing and implementing these models requires significant investment and expertise.
Steps to Ensure Data Privacy in AI Diagnostics
Implement Robust Data Encryption
One of the first steps in ensuring data privacy in AI diagnostics is to implement robust data encryption techniques. Encryption converts data into a coded format that can only be accessed by authorized individuals with the correct decryption key. This ensures that even if data is intercepted, it remains unreadable and secure.
Healthcare organizations should use advanced encryption standards, such as AES-256, to protect patient data. Additionally, encryption should be applied to data both at rest and in transit to provide comprehensive protection. Regularly updating encryption protocols and conducting security audits can further enhance data security.
Adopt Data Anonymization Techniques
Data anonymization is another critical step in protecting patient privacy. Anonymization involves removing or altering personally identifiable information (PII) from datasets, making it impossible to trace the data back to individual patients. This allows AI systems to use the data for training and analysis without compromising patient privacy.
There are various techniques for data anonymization, including data masking, pseudonymization, and generalization. Healthcare organizations should choose the most appropriate method based on their specific needs and the type of data they handle. Regularly reviewing and updating anonymization techniques can help ensure that patient data remains protected.
Ensure Regulatory Compliance
Ensuring compliance with data privacy regulations is essential for protecting patient data in AI diagnostics. Healthcare organizations must stay updated on relevant regulations, such as GDPR and HIPAA, and implement policies and procedures to meet these requirements. This includes obtaining patient consent, conducting regular audits, and maintaining detailed records of data handling practices.
Working with legal experts can help organizations navigate the complexities of data privacy laws and ensure that their AI systems comply with all necessary regulations. Additionally, organizations should invest in ongoing staff training to ensure that all employees understand and adhere to data privacy policies.
FAQs
What is the importance of data privacy in AI diagnostics?
Data privacy is crucial in AI diagnostics because it protects sensitive patient information from unauthorized access and misuse. Ensuring data privacy builds trust in AI technologies and enables the development of effective diagnostic tools.
How can healthcare organizations ensure data privacy in AI diagnostics?
Healthcare organizations can ensure data privacy by implementing robust encryption techniques, adopting data anonymization methods, and ensuring compliance with data privacy regulations. Regular audits and staff training are also essential for maintaining data security.
What are the challenges in balancing data access and privacy?
Balancing data access and privacy is challenging because AI diagnostics require large datasets to function effectively. Overly restrictive data access policies can hinder AI development, while lax policies can compromise patient privacy. Innovative solutions like generative data can help address this challenge.
What role do regulations like GDPR and HIPAA play in data privacy?
Regulations like GDPR and HIPAA provide frameworks for protecting patient data by setting strict guidelines on data handling. Compliance with these regulations is essential for ensuring data privacy and avoiding legal repercussions.
Future of Patient Data Privacy in AI Diagnostics
The future of patient data privacy in AI diagnostics is evolving rapidly, driven by advancements in technology and increasing awareness of data privacy issues. Here are five predictions for the future:
- Enhanced Data Encryption Techniques: Future advancements in encryption technology will provide even more robust protection for patient data, making it increasingly difficult for unauthorized individuals to access sensitive information.
- Widespread Adoption of Generative Data: Generative data models will become more prevalent, allowing AI systems to train on synthetic data that mimics real patient data without compromising privacy.
- Stricter Data Privacy Regulations: Governments worldwide will implement stricter data privacy regulations, ensuring that healthcare organizations adhere to the highest standards of data protection.
- Increased Use of Blockchain Technology: Blockchain technology will be increasingly used to secure patient data, providing a transparent and tamper-proof method of data storage and sharing.
- Greater Emphasis on Patient Consent: There will be a greater emphasis on obtaining and maintaining patient consent for data use, with more advanced systems for managing and tracking consent.
More Information
- Reuters – Data Privacy in AI Healthcare: An article discussing the legal aspects of data privacy in AI healthcare.
- BMC Medical Ethics – Privacy and AI: A detailed exploration of the challenges in protecting health information in the era of AI.
- Frontiers – Balancing Health Data Privacy and Access: An article discussing the ethical considerations of balancing data privacy and access in healthcare AI.
Disclaimer
This is an AI-generated article created for educational purposes. It does not intend to provide advice or recommend specific implementations. The goal is to inspire readers to research and delve deeper into the topics covered.
- The Agentic Startup Manifesto - June 8, 2025
- Remote Hiring in 2025 - April 5, 2025
- Burnout in Remote Teams: How It’s Draining Your Profits - January 27, 2025