Predictive analytics revolutionizes preventive healthcare by enabling early detection and intervention, ultimately improving patient outcomes and reducing costs. This article explores the key aspects of predictive analytics in preventive healthcare, the challenges faced by the industry, and practical solutions to overcome these challenges.
Understanding Predictive Analytics in Preventive Healthcare
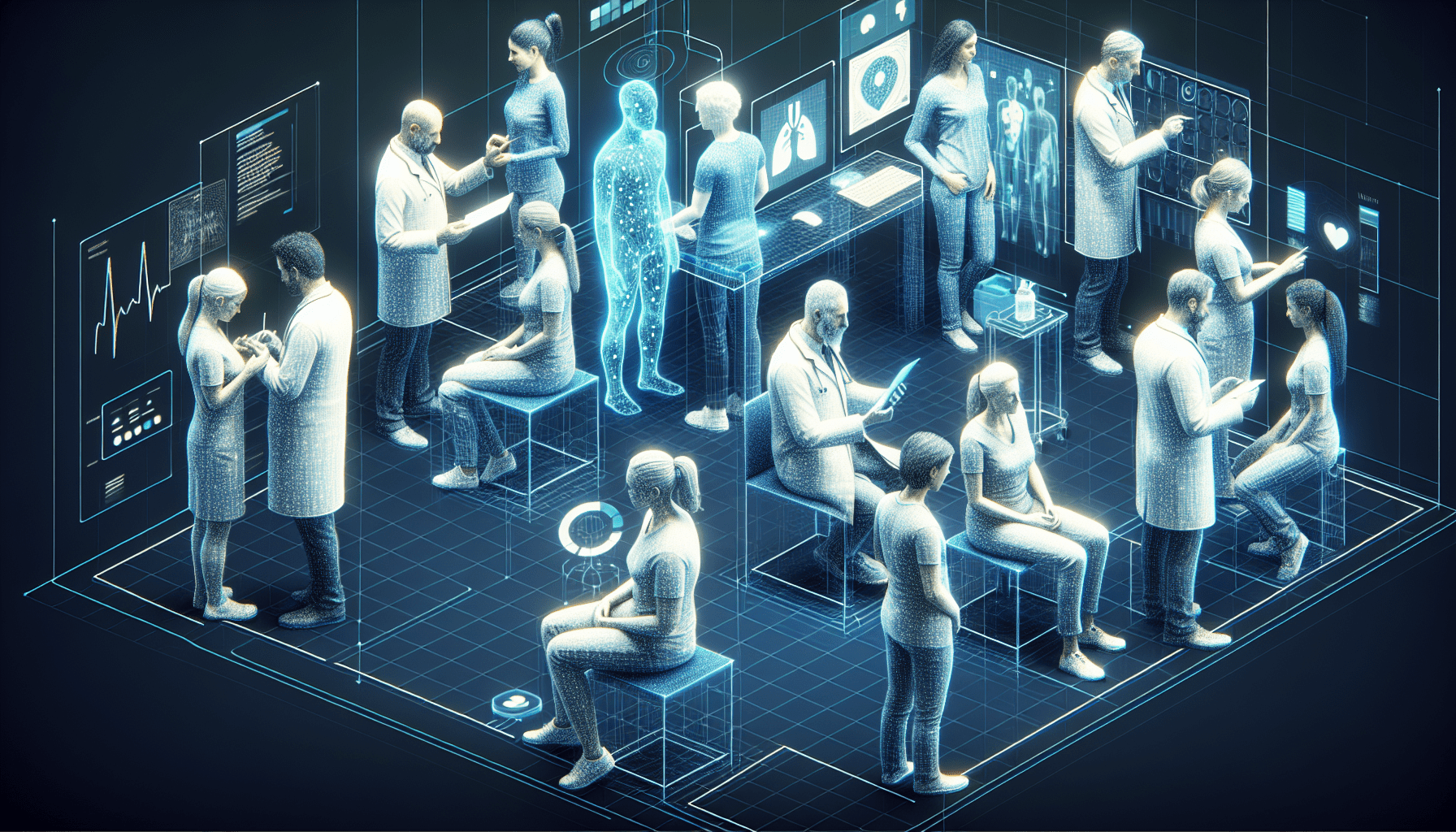
Key Points
- Predictive analytics helps in early detection and intervention.
- It improves patient outcomes and reduces healthcare costs.
- Challenges include data management and integration.
- Solutions involve advanced algorithms and AI integration.
- Future trends point towards more personalized healthcare.
Definition and Importance
Predictive analytics uses historical data, machine learning, and statistical algorithms to predict future outcomes. Preventive healthcare plays a crucial role in identifying potential health issues before they become severe. By analyzing patterns and trends in patient data, healthcare providers can offer timely interventions, reducing the risk of complications and improving overall health outcomes.
The importance of predictive analytics in preventive healthcare cannot be overstated. It allows for a proactive approach to health management, shifting the focus from treatment to prevention. This not only enhances patient care but also significantly reduces healthcare costs by minimizing the need for expensive treatments and hospitalizations.
Moreover, predictive analytics supports personalized medicine, tailoring healthcare plans to individual patients based on their unique risk factors and health history. This personalized approach ensures that patients receive the most effective preventive care, further improving outcomes and patient satisfaction.
Applications in Healthcare
Predictive analytics has a wide range of applications in preventive healthcare. One of the most significant is in the early detection of chronic diseases such as diabetes, heart disease, and cancer. By analyzing patient data, predictive models can identify individuals at high risk of developing these conditions, allowing for early intervention and lifestyle modifications to prevent disease onset.
Another critical application is in hospital readmission prevention. Predictive analytics can identify patients at risk of readmission, enabling healthcare providers to implement targeted interventions to reduce this risk. This not only improves patient outcomes but also helps hospitals avoid financial penalties associated with high readmission rates.
Additionally, predictive analytics can enhance population health management by identifying trends and patterns in large datasets. This allows healthcare organizations to develop targeted public health initiatives and allocate resources more effectively, ultimately improving the health of entire communities.
Challenges in the Health Technology Industry
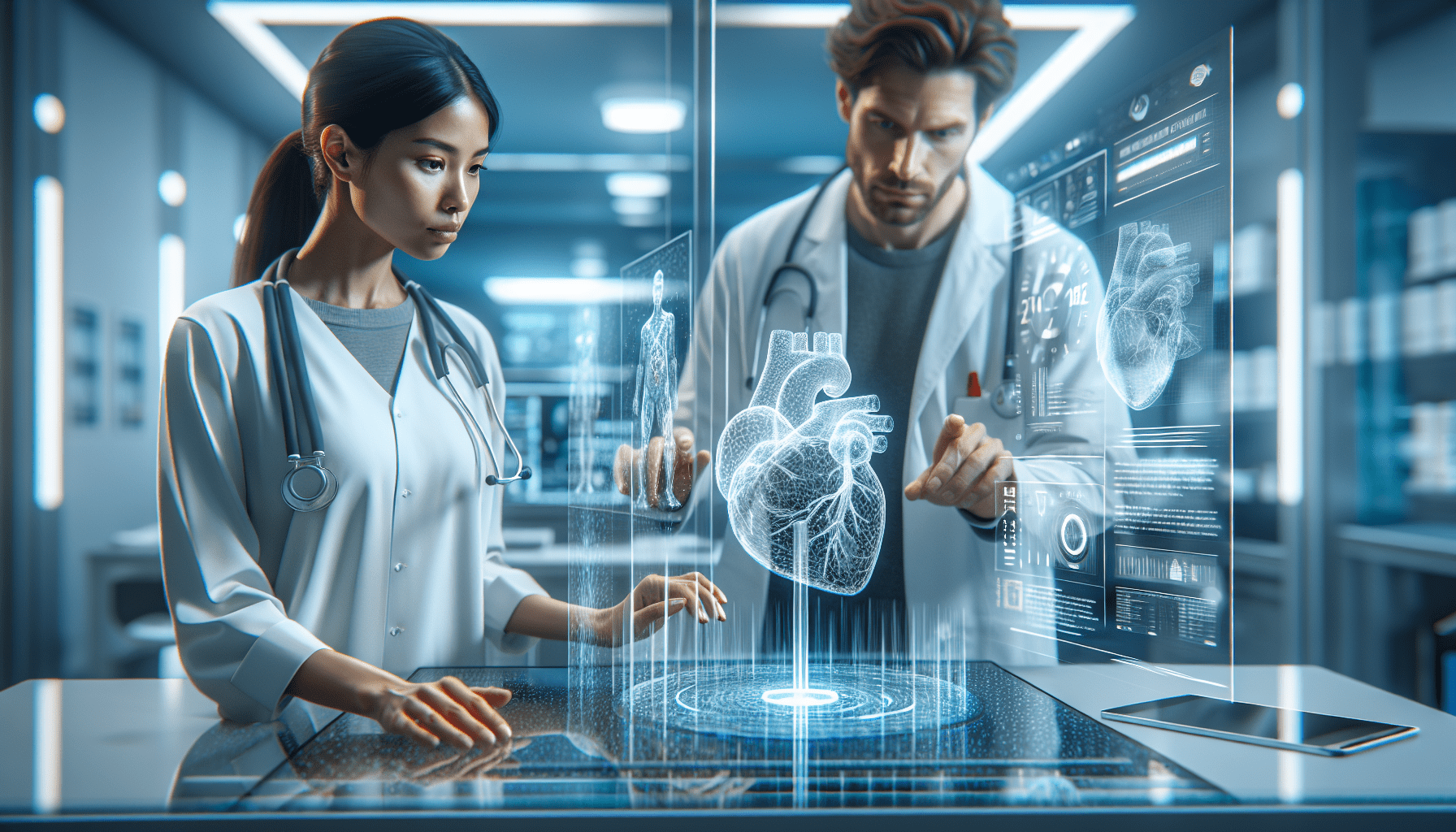
- Data Management Issues:
One of the most significant challenges in the health technology industry is inefficient health data management. Healthcare organizations generate vast amounts of data daily, but managing and analyzing this data effectively remains a daunting task. The data is often unstructured, heterogeneous, and stored in disparate systems, making it difficult to integrate and analyze.
This lack of efficient data management hinders the ability to leverage predictive analytics fully. Without accurate and comprehensive data, predictive models cannot provide reliable insights, limiting their effectiveness in preventive healthcare. Addressing these data management issues is crucial for the successful implementation of predictive analytics in the industry.
- Lack of Telemedicine Integration:
Another challenge is the lack of telemedicine integration. Telemedicine has become increasingly important, especially in the wake of the COVID-19 pandemic. However, many healthcare organizations struggle to integrate telemedicine with their existing systems and workflows. This lack of integration can lead to fragmented care and missed opportunities for preventive interventions.
Integrating telemedicine with predictive analytics can enhance preventive healthcare by providing real-time data and enabling remote monitoring of patients. This allows healthcare providers to identify potential health issues early and intervene promptly, improving patient outcomes and reducing the need for in-person visits.
- Need for AI in Diagnostics:
The need for AI in diagnostics is another pressing challenge. Traditional diagnostic methods can be time-consuming and prone to errors, leading to delayed or incorrect diagnoses. AI-powered diagnostic tools can significantly improve the accuracy and speed of diagnoses, enabling timely interventions and better patient outcomes.
However, implementing AI in diagnostics requires significant investment in technology and training. Healthcare organizations must overcome these barriers to fully harness the potential of AI in predictive analytics and preventive healthcare.
Implementing Predictive Analytics in Preventive Healthcare
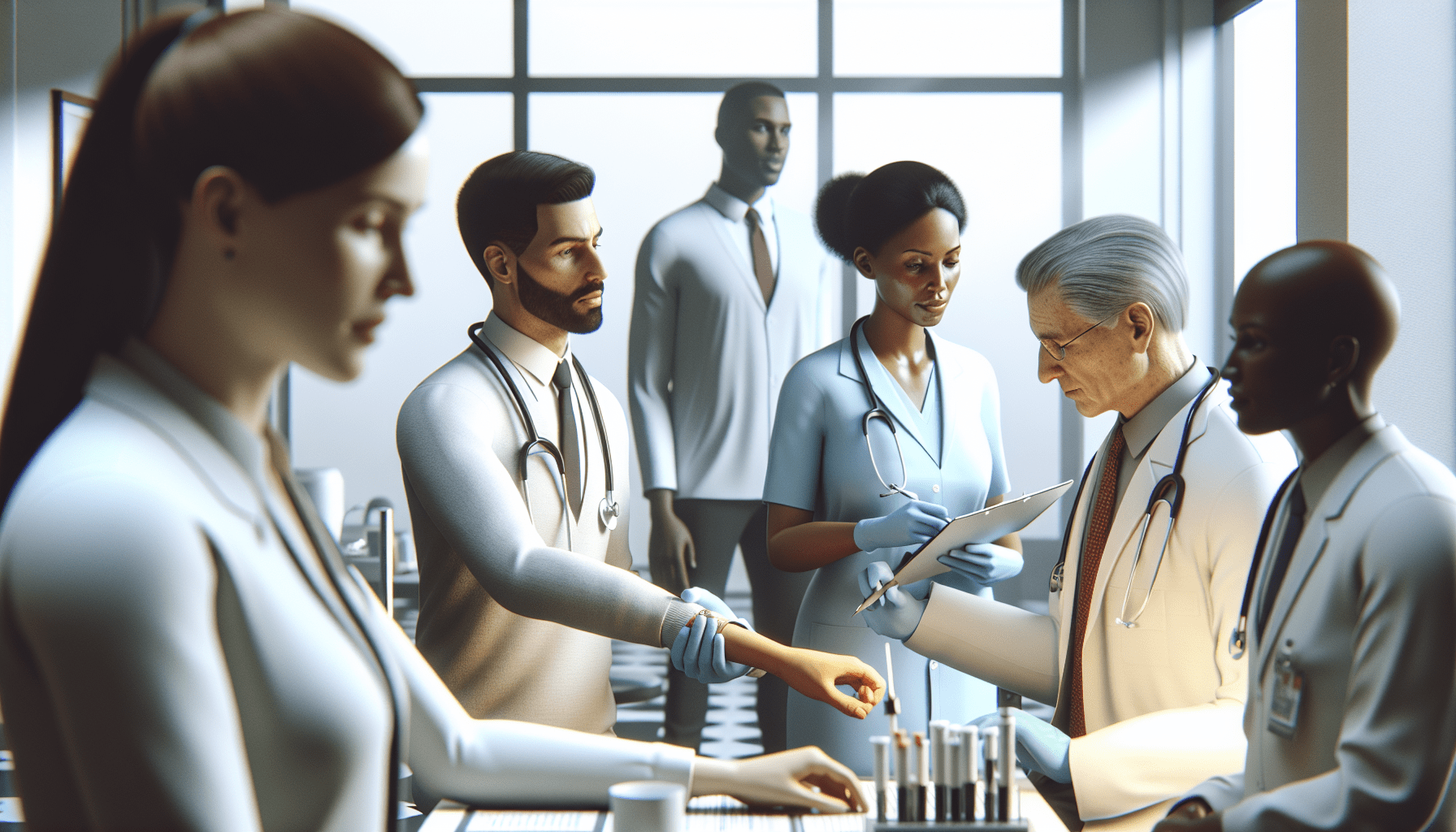
Step 1: Data Collection and Integration
The first step in implementing predictive analytics in preventive healthcare is data collection and integration. Healthcare organizations must gather data from various sources, including electronic health records (EHRs), wearable devices, and telemedicine platforms. This data must be integrated into a centralized system to provide a comprehensive view of each patient’s health.
Effective data integration requires robust data management practices and the use of advanced technologies such as data lakes and cloud-based platforms. These technologies enable healthcare organizations to store and analyze large volumes of data efficiently, providing the foundation for predictive analytics.
Step 2: Developing Predictive Models
Once the data is collected and integrated, the next step is to develop predictive models. These models use machine learning algorithms to analyze historical data and identify patterns and trends. The models can then predict future health outcomes and identify individuals at risk of developing specific conditions.
Developing accurate predictive models requires collaboration between data scientists, healthcare professionals, and IT experts. This multidisciplinary approach ensures that the models are based on sound medical knowledge and are tailored to the specific needs of the healthcare organization.
Step 3: Implementing and Monitoring Interventions
The final step is to implement and monitor interventions based on the insights provided by the predictive models. Healthcare providers can use these insights to develop personalized preventive care plans for each patient, addressing their unique risk factors and health needs.
Monitoring the effectiveness of these interventions is crucial for continuous improvement. Healthcare organizations should regularly review the outcomes of their preventive care programs and adjust their strategies as needed to ensure optimal results.
Future of Predictive Analytics in Preventive Healthcare
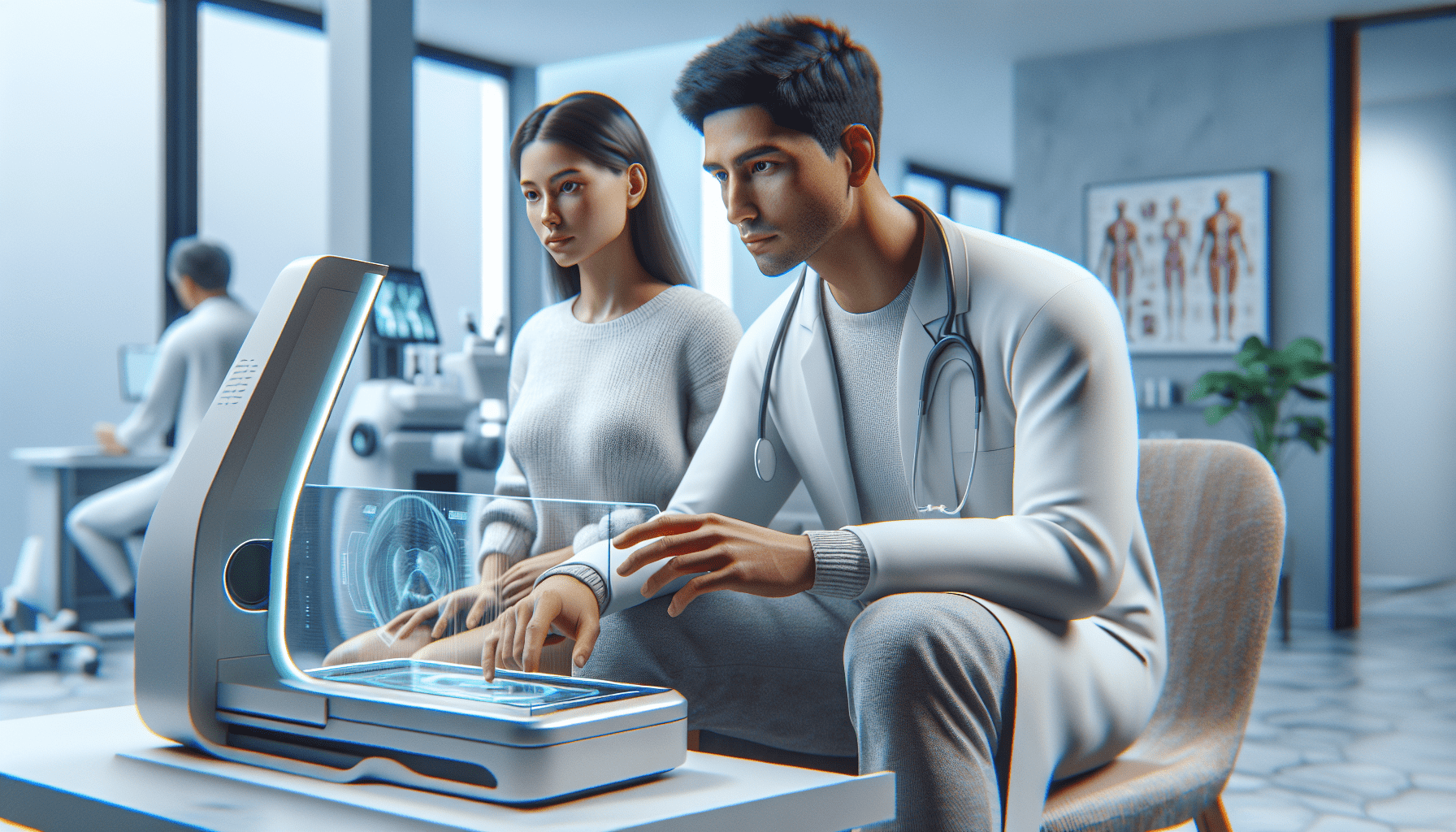
The future of predictive analytics in preventive healthcare looks promising, with several trends and advancements on the horizon. Here are five predictions for the future:
- Increased Personalization: Predictive analytics will enable more personalized healthcare plans, tailored to individual patients’ unique risk factors and health history.
- Integration with Telemedicine: The integration of predictive analytics with telemedicine will enhance remote monitoring and real-time data collection, improving preventive care.
- Advancements in AI: AI-powered diagnostic tools will become more prevalent, improving the accuracy and speed of diagnoses and enabling timely interventions.
- Improved Data Management: Advances in data management technologies will enable more efficient storage and analysis of healthcare data, providing a solid foundation for predictive analytics.
- Focus on Population Health: Predictive analytics will play a crucial role in population health management, helping healthcare organizations develop targeted public health initiatives and allocate resources more effectively.
More Information
- Big data analytics for preventive medicine | NIG: This article discusses the role of big data analytics in preventive medicine and its impact on healthcare.
- How Big Data Analytics Can Support Preventive Health | Health IT Analytics: This source explores how big data analytics can support preventive healthcare and improve patient outcomes.
- Preventive Care: How Big Data, Analytics, AI & More Improve Outcomes | HealthTech: This article highlights the benefits of big data, analytics, and AI in preventive care and their role in improving healthcare outcomes.
Disclaimer
This is an AI-generated article with educative purposes and doesn’t intend to give advice or recommend its implementation. The goal is to inspire readers to research and delve deeper into the topics covered in the article.
- Burnout in Remote Teams: How It’s Draining Your Profits - January 27, 2025
- Signs You’re Understaffed - January 20, 2025
- The Cost of Silence: Communicating Negative Feedback - January 13, 2025