The adoption of AI for content generation in early-stage startups can be seen as a double-edged sword. While it offers the promise of efficiency and scalability, the initial and ongoing costs can vary widely. These include expenses related to software acquisition, customization, training, and potential increases in computational resources.
Furthermore, some AI tools require significant amounts of data to function effectively, which can be a challenge for startups that haven’t yet established a large customer base. However, many startups can leverage cost-effective freemium models or pay-as-you-go options offered by various AI content generation platforms. These options allow startups to experiment with the technology and scale their usage as their needs evolve, mitigating the initial financial burden.
What initial investments are needed for AI content generation?
Startups delving into AI content generation face upfront costs. These include acquiring the software itself, either through a subscription or a one-time purchase. Additionally, integrating the tool with existing systems might require expert assistance from data scientists or developers.
Furthermore, some AI tools require a significant amount of training data to function effectively, which can necessitate additional investment in data acquisition or creation. However, exploring freemium models, pay-as-you-go services, or even open-source tools can help startups mitigate these initial investments and experiment with AI content generation before fully committing.
How does training and customization impact costs?
Customizing AI to produce content that aligns with a startup’s specific brand voice and requirements can necessitate significant training in the AI model. This could include costs for data acquisition, annotation, and possibly hiring data scientists to oversee the training process.
The complexity of the training process also impacts cost. Simpler customizations requiring brand-specific keywords or style guidelines might be achievable with in-house tools and expertise. However, for in-depth customization aiming to capture the nuances of complex brand voice or highly technical content, advanced techniques and potentially more powerful computing resources may be needed, further increasing the cost.
Are there ongoing costs associated with AI content generation?
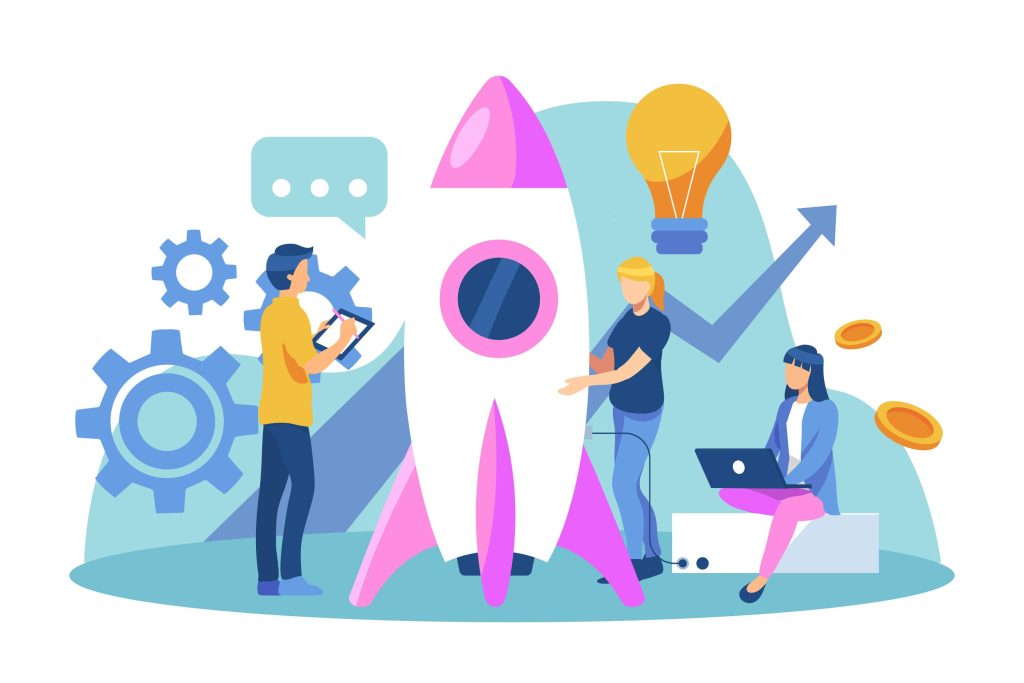
Beyond initial setup, AI systems require maintenance and continuous training to stay updated with the latest language models and company needs. Additionally, as usage increases, so does the demand for computational resources, potentially leading to higher cloud computing fees.
Furthermore, some platforms might charge per generated content piece, meaning your ongoing costs will directly correlate with your content output. This pay-as-you-go structure can be beneficial for startups with fluctuating content needs, but it’s crucial to factor in potential cost increases as your content generation ramps up.
How can startups minimize these costs?
To minimize costs, startups should explore AI content generation platforms offering scalable pricing models. Leveraging open-source tools or seeking partnerships for data and resources can also reduce expenses. Carefully planning the extent of customization and scaling usage as the business grows will help control costs.
It’s important to remember that AI-generated content isn’t a magic bullet. While it excels at producing large amounts of content quickly, it often lacks the human touch and nuanced creativity that truly resonates with audiences. To bridge this gap, startups should establish a clear editorial process. This could involve using human editors to refine AI-generated content, ensuring brand consistency, and injecting a personal voice. By combining AI’s efficiency with human expertise, startups can achieve cost-effective content creation that fosters engagement.
- Analyzing Patterns in Failed Products - July 25, 2024
- Hybrid Cryptographic Systems - July 24, 2024
- Inadequate Threat Intelligence Integration - July 23, 2024