CycleGAN is a type of generative adversarial network that has gained recognition. It excels at converting images from one style to another without paired training data.
This innovative technology finds applications in various real-world scenarios, including content creation in social media. Additionally, it aids automotive companies in synthesizing sensor data for autonomous vehicles.
CycleGAN proves particularly effective in situations requiring the generation of large datasets that mirror real-world diversity. This is achieved without the high costs and time consumption typically associated with traditional data collection methods.
How is CycleGAN utilized in content creation for social media?
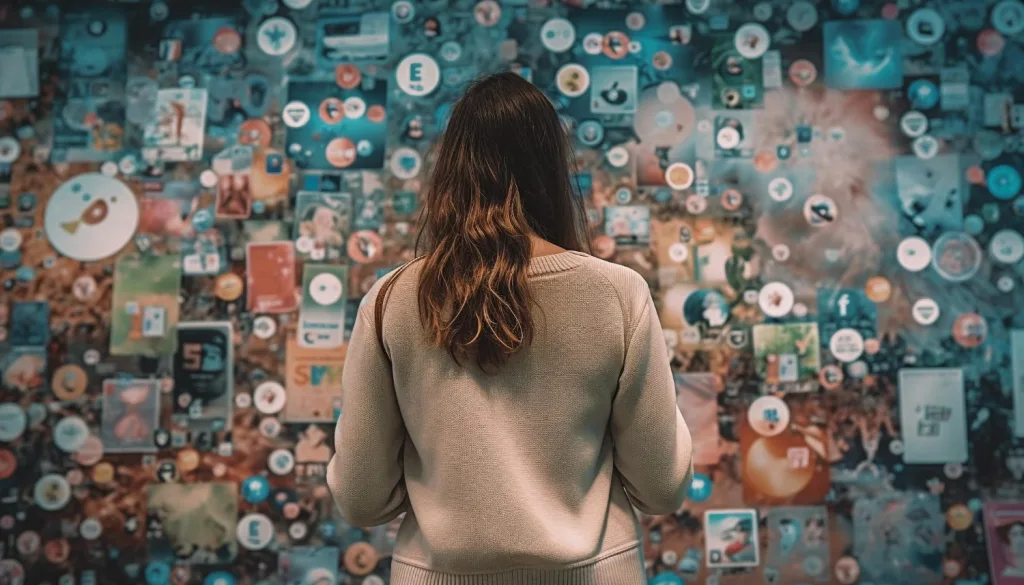
In the realm of social media, content creators always strive to be unique. CycleGAN stands out as a game-changer, allowing them to transform ordinary images into captivating art-style visuals.
For example, converting real-world photos into famous painters’ styles creates distinctive profile pictures or eye-catching posts, enhancing visual appeal and expressing creativity.
Tech-focused marketing teams also benefit from CycleGAN, automating and personalizing content for audiences. This includes generating photo-realistic scenes for product visualization and creating virtual avatars for brand representation.
These applications help create visually rich content, connecting with diverse audiences and boosting conversion rates for businesses in tech hubs.
Can CycleGAN enhance medical image analysis?
Yes, CycleGAN has a promising application in the medical field, particularly in medical image analysis. It can convert medical images from one modality to another, for example, from MRI to CT scans, addressing insufficient datasets.
This improves diagnostic tool training, aiding health professionals in making informed decisions with a comprehensive view of available medical images.
The potential of CycleGAN in medical imaging also extends to improving the visualization of diseases. It can assist in highlighting certain conditions that might be less visible in one imaging modality compared to another.
This sophisticated image transformation helps in early detection and better monitoring of diseases, ultimately leading to improved patient outcomes.
In what ways does CycleGAN contribute to fashion and retail innovation?
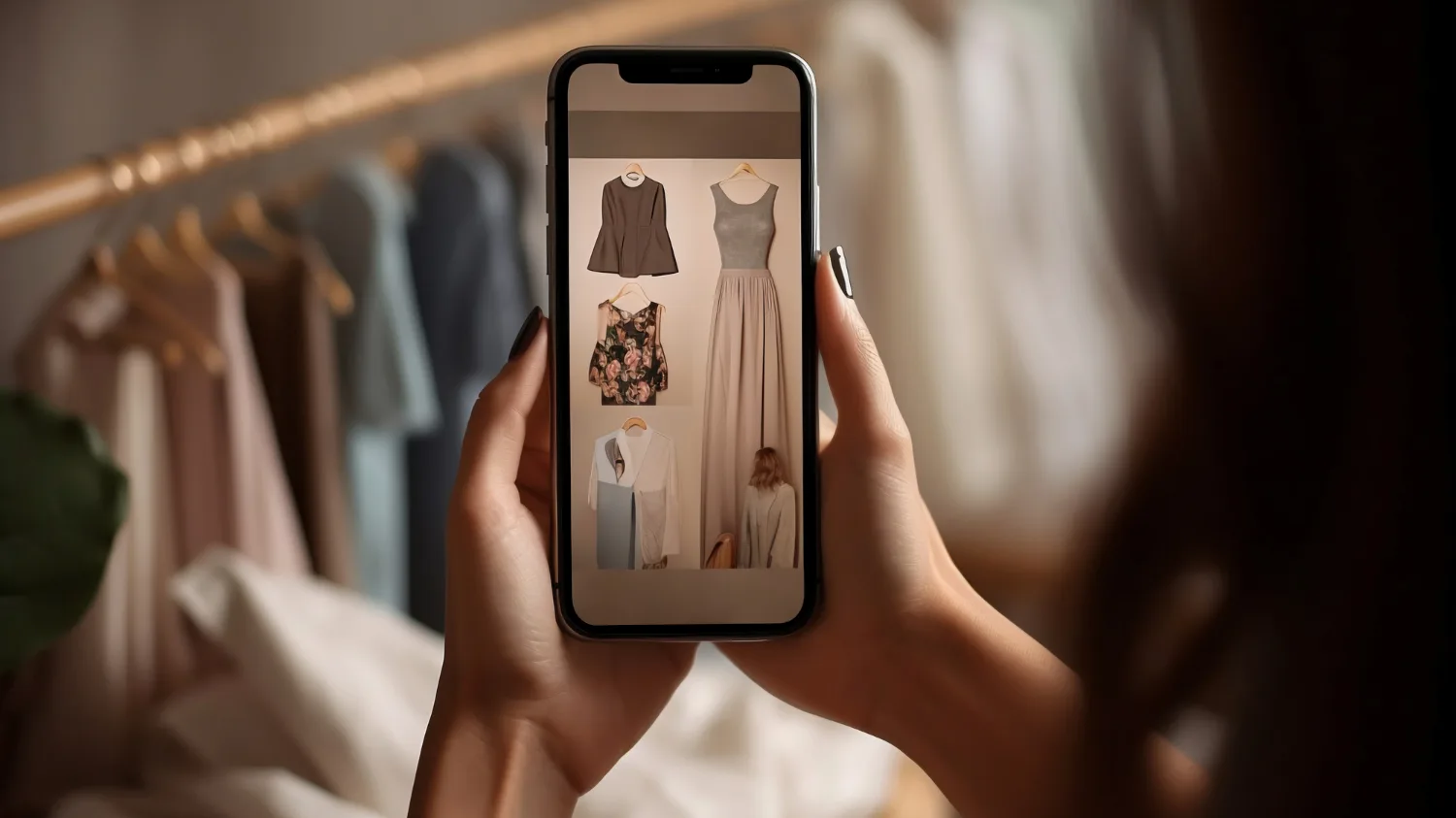
Fashion and retail industries are using CycleGAN to revolutionize online shopping.
This AI allows presenting a single clothing piece in various textures and styles without the need for multiple photoshoots, saving time and expenses.
CycleGAN’s ability to simulate realistic lighting and textures aids in creating an authentic online shopping experience.
Beyond display, it assists in trend prediction and design, reducing the gap between customer demand and inventory, and addressing overproduction issues in the fashion industry.
How does CycleGAN benefit the automotive industry, especially in autonomous driving?
In autonomous vehicle development, generating synthetic sensor data is key.
CycleGAN is crucial, converting camera images into data resembling various sensors like LIDAR or radar. Developers use this synthetic data to train autonomous systems in a controlled environment, expediting development and ensuring safety before deployment on roads.
CycleGAN also aids in simulating diverse driving conditions, helping autonomous systems learn to react to various scenarios, including rare or challenging ones. This contributes to resilient and intelligent driving algorithms, vital for the reliability and public acceptance of autonomous vehicles.
Conclusion
The versatility of CycleGAN is showcased through its myriad of applications in different sectors.
From creating striking visuals for social media to enhancing online shopping experiences in fashion and retail, from facilitating medical diagnostics to accelerating the development of autonomous vehicles, CycleGAN is a revolutionary tool.
It addresses the pressing need for automated and robust content creation and innovation, serving as a cornerstone for data-driven decision-making in modern tech enterprises. For a deeper understanding of how CycleGAN functions, refer to this functional overview.
Its ability to perform unpaired image-to-image translation is creating new possibilities and solving intricate problems that were challenging with traditional techniques.
Its relevance in the technologically advanced clusters of Silicon Valley, New York, London, Berlin, and Bangalore underlines the importance of continuing to explore and apply this adaptable AI technology for practical and impactful solutions across industries. 🌍🔍🚀
- Analyzing Patterns in Failed Products - July 25, 2024
- Hybrid Cryptographic Systems - July 24, 2024
- Inadequate Threat Intelligence Integration - July 23, 2024