For those with an eye for the ways technology shapes the future, the term processing carries significant weight, particularly within the field of Generative Artificial Intelligence (AI).
This article is not merely a resource; it is a navigation tool designed to elucidate the intricate processes underlying Generative AI. As this technology becomes increasingly pivotal for innovation in various sectors, comprehending its processes is invaluable.
Identifying and deploying processing techniques effectively can be transformative for product development and strategic planning. Consequently, this knowledge can catalyze new directions in the creation of digital content, algorithmic decision-making, and beyond.
Understanding Generative AI
Defining Generative AI
At its core, Generative AI refers to the subset of artificial intelligence focused on creating new content, be it text, images, or even code. Essentially, it operates by understanding patterns and generating novel outputs that reflect learned data structures.
Companies like OpenAI with their GPT-3 or DALL-E are flagship examples, producing results indistinguishable from those made by humans. These technologies promise to shape how businesses approach tasks that once required significant human effort.
The Role of Machine Learning
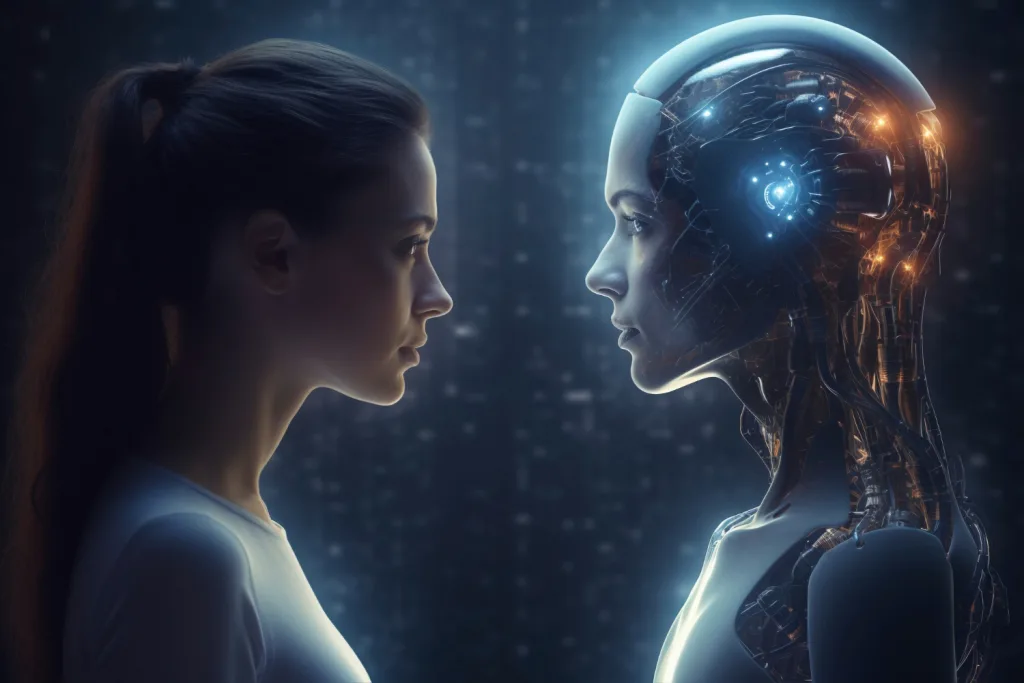
Machine learning is the driving force behind the sophistication of Generative AI. By training models on vast datasets, the AI learns to emulate patterns and structures, allowing for the generation of new, similar pieces of content.
From Google’s DeepMind to smaller start-ups, organizations are leveraging machine learning to process data and evolve their Generative AI capabilities, optimizing decision-making and creative processes.
Key Components of Processing
Delving deeper, processing in Generative AI involves several key components: data preparation, algorithm selection, model training, and validation. Each stage ensures the system functions with precision and relevance.
Proper data processing and algorithm application are what make tools like IBM’s Watson so adept at providing solutions across industries, from healthcare diagnostics to financial predictions.
Processing Techniques
Variants like supervised, unsupervised, and reinforcement learning offer different approaches for the AI to process information. Techniques are chosen based on the nature of the task and the intended outcome.
Whether it’s Nvidia’s use of GPU processing for faster computation or Adobe’s Sensei platform enhancing creative workflows, the processing technique determines efficiency and effectiveness.
Designing the AI’s Learning Process
Curating Training Data
The carefully curated datasets that AIs learn from ideally represent a wide range of scenarios to which the AI will respond. Consequently, this ensures a comprehensive understanding and aptitude to generate diverse content.
Moreover, platforms like Twitter and Pinterest use such curation to fine-tune their algorithms, offering content recommendations that keep users engaged and satisfied with their personal feeds.
Choosing the Right Model
Model selection is critical; the right model harnesses the patterns in data effectively. Decisions made here can save costs and time while improving output quality.
Amazon’s forays into AI model development attest to this, balancing customer personalization with operational efficiency.
Training and Refinement
Effective training includes error detection and rectification, helping the AI evolve and align more closely with objectives. Continuous refinement allows for more accurate and creative output over time.
Spotify’s recommendation algorithms demonstrate this iterative process well, constantly adapting to user preferences to provide a personally tailored listening experience.
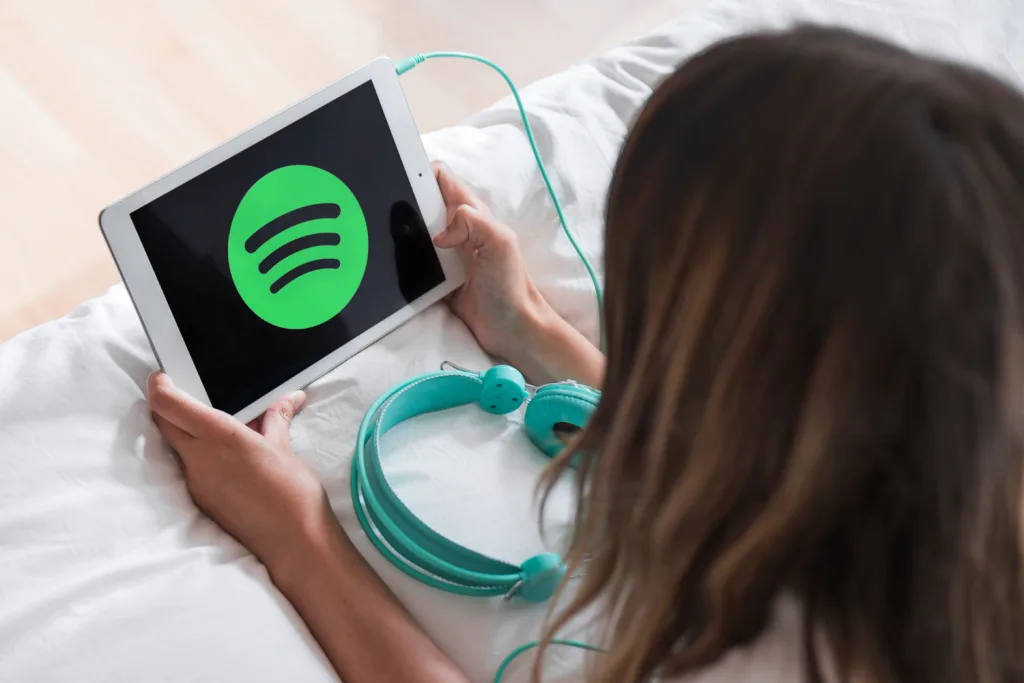
Validation and Testing
Successful generative models must be validated against unbiased data, ensuring their reliability. This stage is as much about confirming functionality as it is about fine-tuning performance.
Autonomous vehicle technologies, like those developed by Tesla, are continuously tested and validated in real-world conditions to ensure safety and efficiency.
Integration and Application of Generative AI
In Content Creation
Generative AI finds robust applications in content creation, where it can automate and personalize content at scale. In marketing, for instance, this can revolutionize campaign strategies.
Furthermore, the AI-generated artworks that have begun to appear at auctions exemplify this potential, blurring the lines between human and machine creativity.
In Decision-Making
Data-driven decision-making is another arena where Generative AI excels. By simulating multiple outcomes, it helps leaders make more informed choices, with less risk and higher potential returns.
Financial institutions utilize such technologies for risk assessment and market predictions, often with startling accuracy.
In Innovation and Product Design
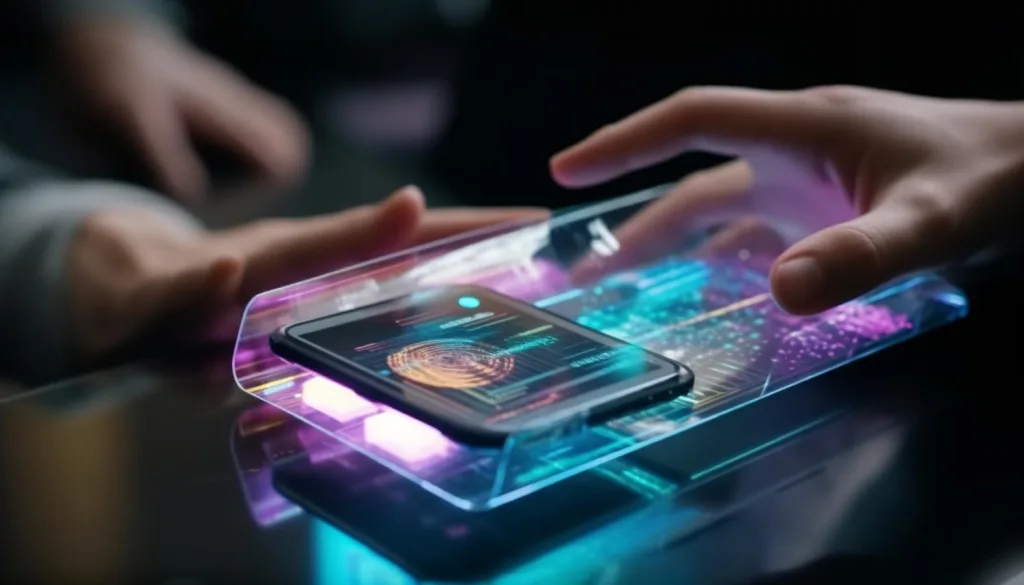
The influence of Generative AI in product design is growing, where its ability to envision numerous prototypes can expedite innovation and reduce resource wastage.
Adidas, for example, uses AI to create virtual prototypes, allowing for rapid experimentation with design before any physical product is made.
Customizing User Experiences
User experience customization is the final link, ensuring products and services align with specific user needs and preferences. AI helps tailor experiences, retaining user engagement effectively.
Netflix‘s content suggestion algorithms are a testament to this, curating viewer experiences and maintaining high platform engagement levels.
Assessing the Impact of Generative AI on Industries
Effect on the Creative Industry
The creative sector is undergoing a transformation as Generative AI removes traditional barriers and automates part of the creative process. This allows for new forms of storytelling and art creation that were once unthinkable.
Implications for Businesses
Businesses across sectors are finding that Generative AI can automate routine tasks and generate insights, leading to more strategic and inventive ventures.
This is evident in CRM platforms like Salesforce, where AI is used to predict customer behaviors and optimize sales processes.
Challenges and Ethical Considerations
While the benefits are numerous, challenges such as ensuring data privacy, avoiding biases, and maintaining ethical standards are critical in the application of Generative AI.
This conversation is vital, as expressed by leading tech ethicists and legislators deliberating over the best courses of action for AI governance.
Trends and Future Outlook
The future of Generative AI looks bright, with ongoing advancements promising ever more nuanced applications and astonishing capabilities.
As luminaries like Elon Musk and Jeff Bezos invest in AI ventures, their vision of its potential signifies an area of rapid growth and development.
Conclusion
The intricacies of processing in Generative AI remain a substantial undertone in the melody of technological progress.
From fundamental principles to hands-on implementation, understanding this core concept bears the potential to spur a cascade of developments in various industries.
This exploration offers more than knowledge—it is a beacon for innovation, beckoning to those who seek to refine the interplay between technology and human creativity.
The embrace of this technology, when guided by thoughtful consideration of its capabilities and implications, sets the stage for not only adapting to the digital landscape but also shaping it.
As processing techniques evolve and integrations become more seamless, Generative AI stands poised to redefine what is possible in automation, personalized experiences, and creative expression.
- Remote Hiring in 2025 - April 5, 2025
- Burnout in Remote Teams: How It’s Draining Your Profits - January 27, 2025
- Signs You’re Understaffed - January 20, 2025