Predictive analytics for inventory management is a game-changer for businesses looking to optimize their stock levels, reduce costs, and improve operational efficiency.
By leveraging historical data and advanced algorithms, companies can accurately forecast demand and make informed decisions about their inventory.
Understanding Predictive Analytics for Inventory Management
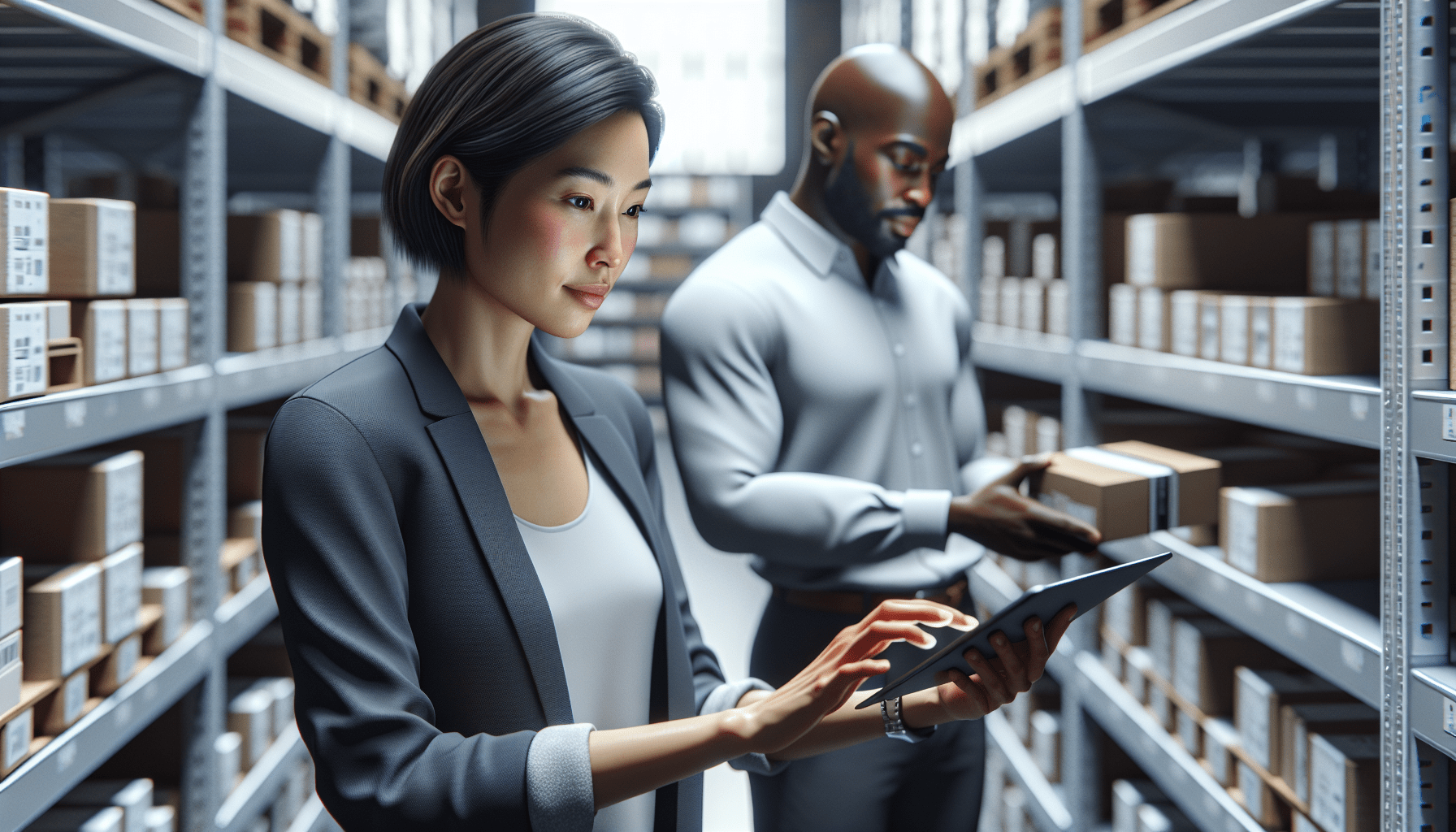
Key Points
- Accurate demand forecasting helps prevent stockouts and overstock situations.
- Reduction in holding costs by optimizing inventory levels.
- Enhanced supplier relationships through better planning and collaboration.
- Improved customer satisfaction by ensuring product availability.
- Data-driven decision-making for better operational efficiency.
What is Predictive Analytics?
Predictive analytics involves using historical data, statistical algorithms, and machine learning techniques to identify the likelihood of future outcomes based on past data. In the context of inventory management, it helps businesses forecast demand, optimize stock levels, and reduce costs.
By analyzing patterns and trends in sales data, predictive analytics can provide insights into future demand. This allows businesses to adjust their inventory levels accordingly, ensuring they have the right amount of stock at the right time.
Predictive analytics also considers external factors such as market trends, seasonal variations, and economic indicators. This comprehensive approach helps businesses make more accurate predictions and improve their overall inventory management strategy.
Benefits of Predictive Analytics in Inventory Management
One of the primary benefits of predictive analytics in inventory management is accurate demand forecasting. By analyzing historical sales data and other relevant factors, businesses can predict future demand with a high degree of accuracy. This helps prevent stockouts and overstock situations, leading to better customer satisfaction and reduced holding costs.
Another significant benefit is the reduction in holding costs. By optimizing inventory levels, businesses can minimize the amount of capital tied up in excess stock. This frees up resources that can be used for other critical business activities.
Predictive analytics also enhances supplier relationships. By providing accurate forecasts, businesses can work more effectively with their suppliers to ensure timely deliveries and avoid disruptions in the supply chain. This leads to stronger, more collaborative relationships with suppliers.
Challenges in the Industry
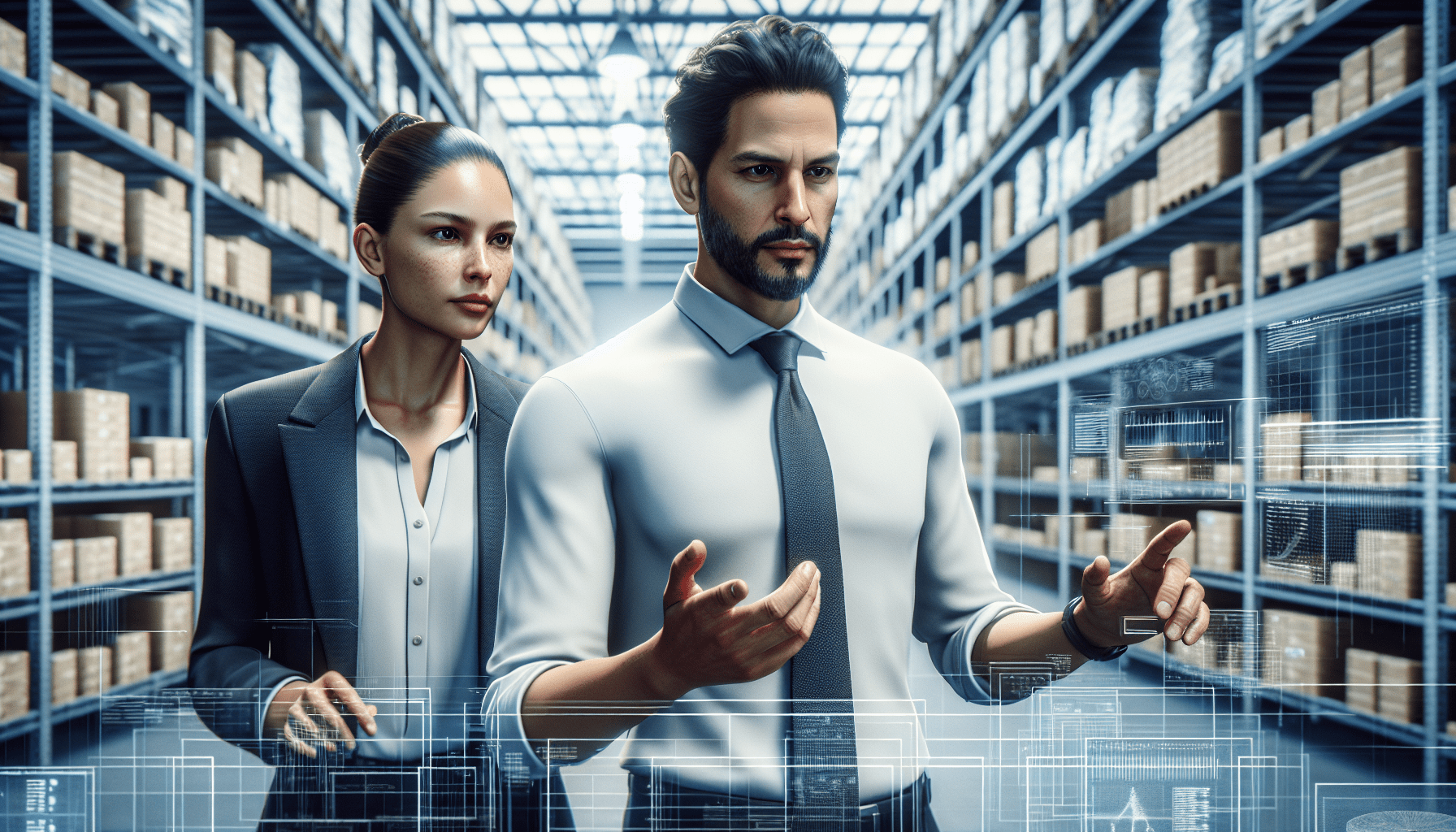
High Operational Costs
One of the most significant challenges in the industry is the high operational costs associated with inventory management. Maintaining optimal stock levels requires substantial investment in storage facilities, personnel, and technology. These costs can quickly add up, especially for businesses with large and diverse inventories.
Additionally, excess inventory ties up valuable capital that could be used for other business activities. This can lead to cash flow problems and limit a company’s ability to invest in growth opportunities.
Inefficiency in Inventory Management
Inefficiency in inventory management is another major challenge. Traditional methods of managing inventory often rely on manual processes and outdated systems. This can lead to errors, delays, and inefficiencies that impact the overall performance of the business.
For example, manual inventory tracking can result in inaccurate data, leading to stockouts or overstock situations. These inefficiencies can also affect customer satisfaction, as products may not be available when needed.
Lack of Innovation
The lack of innovation in inventory management is a significant barrier to improving operational efficiency. Many businesses continue to rely on traditional methods and are hesitant to adopt new technologies. This resistance to change can prevent companies from realizing the full potential of predictive analytics.
Without innovation, businesses may struggle to keep up with the rapidly changing market demands and competitive pressures. This can lead to missed opportunities and a decline in overall performance.
Implementing Predictive Analytics for Inventory Management
Step 1: Data Collection and Integration
The first step in implementing predictive analytics for inventory management is to collect and integrate relevant data. This includes historical sales data, market trends, seasonal variations, and other external factors. By consolidating this data into a single system, businesses can ensure they have a comprehensive view of their inventory.
Data integration is crucial for accurate predictions. Businesses should invest in robust data management systems that can handle large volumes of data and provide real-time insights. This will enable them to make informed decisions based on accurate and up-to-date information.
Step 2: Developing Predictive Models
Once the data is collected and integrated, the next step is to develop predictive models. These models use statistical algorithms and machine learning techniques to analyze the data and identify patterns and trends. By understanding these patterns, businesses can make accurate predictions about future demand.
Developing predictive models requires expertise in data science and analytics. Businesses may need to invest in training their staff or hiring external experts to build and maintain these models. Continuous refinement and validation of the models are also essential to ensure their accuracy and reliability.
Step 3: Implementing and Monitoring the System
After developing the predictive models, the final step is to implement and monitor the system. This involves integrating the models into the existing inventory management system and using them to make data-driven decisions. Businesses should also establish key performance indicators (KPIs) to measure the effectiveness of the system.
Regular monitoring and evaluation are crucial to ensure the system is working as intended. Businesses should continuously review the predictions and adjust their inventory levels accordingly. This will help them stay responsive to changing market demands and maintain optimal stock levels.
FAQs
What is predictive analytics in inventory management?
Predictive analytics in inventory management involves using historical data, statistical algorithms, and machine learning techniques to forecast future demand and optimize stock levels. This helps businesses make informed decisions about their inventory and improve operational efficiency.
How does predictive analytics reduce holding costs?
Predictive analytics reduces holding costs by optimizing inventory levels. By accurately forecasting demand, businesses can minimize excess stock and reduce the amount of capital tied up in inventory. This leads to lower storage costs and frees up resources for other business activities.
What are the benefits of predictive analytics for supplier relationships?
Predictive analytics enhances supplier relationships by providing accurate demand forecasts. This allows businesses to work more effectively with their suppliers, ensuring timely deliveries and avoiding disruptions in the supply chain. Stronger supplier relationships lead to better collaboration and improved overall performance.
What challenges do businesses face in implementing predictive analytics?
Challenges in implementing predictive analytics include data quality issues, the complexity of algorithms, and the need for skilled personnel. Businesses may need to invest in training and technology to overcome these challenges and ensure the accuracy and reliability of their predictive models.
Future of Predictive Analytics in Inventory Management
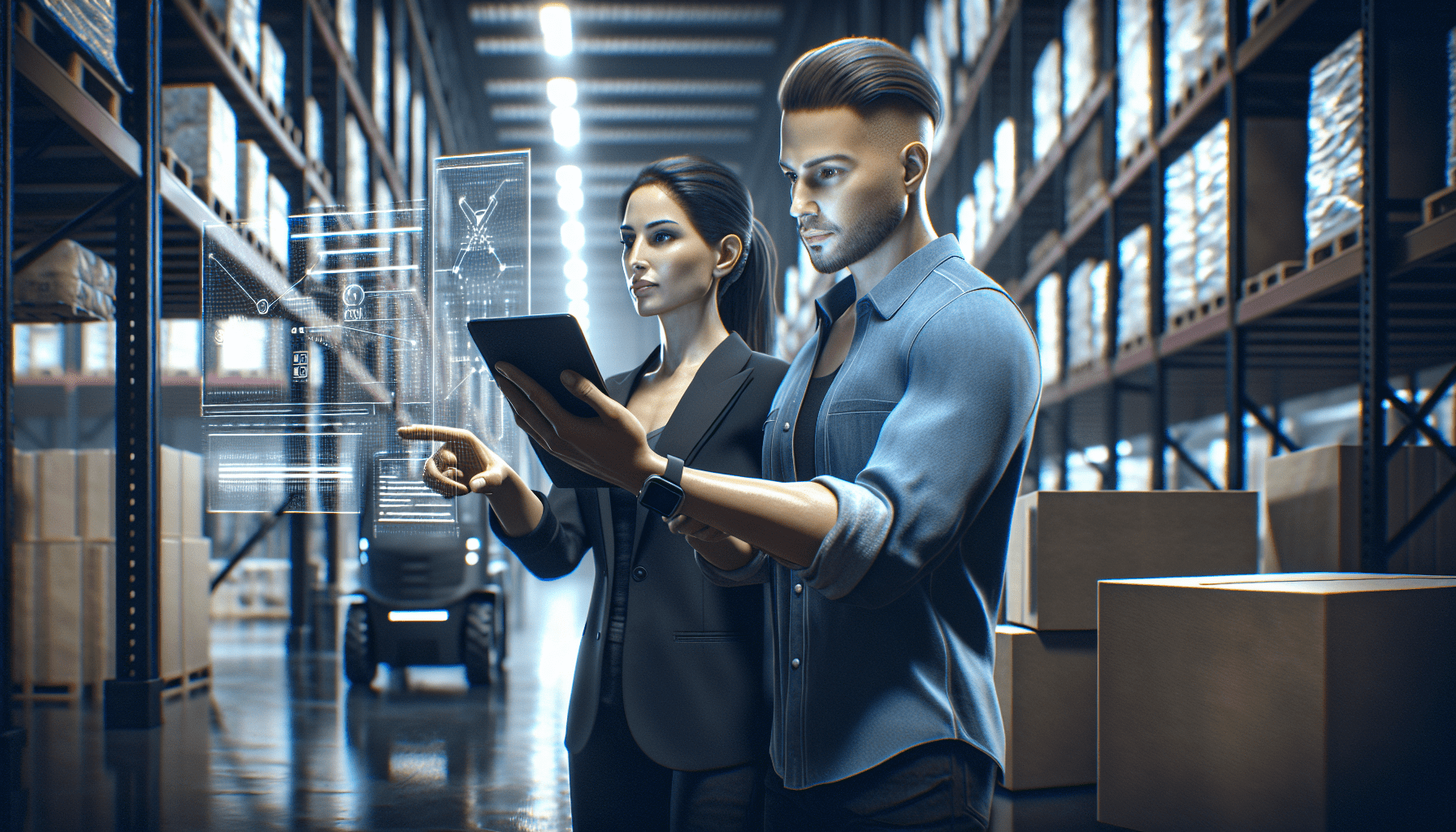
The future of predictive analytics in inventory management looks promising, with several trends and advancements expected to shape the industry. Here are five predictions:
- Increased adoption of AI and machine learning: Businesses will increasingly leverage AI and machine learning to enhance their predictive models and improve accuracy.
- Integration with IoT devices: The integration of predictive analytics with IoT devices will provide real-time data and insights, enabling businesses to make more informed decisions.
- Enhanced data visualization tools: Advanced data visualization tools will help businesses better understand and interpret their data, leading to more effective inventory management strategies.
- Greater focus on sustainability: Predictive analytics will play a crucial role in helping businesses reduce waste and improve sustainability in their inventory management practices.
- Increased collaboration across the supply chain: Predictive analytics will facilitate better collaboration and communication across the supply chain, leading to more efficient and effective inventory management.
More Information
- Leveraging the Power of Inventory Management Predictive Analytics | by Tellius Inc | Medium: An article exploring the significance and benefits of predictive analytics in inventory management.
- The Role of Predictive Analytics in Supply Chain Inventory Management – Supply Chain Technology News: A detailed look at how predictive analytics is transforming supply chain inventory management.
- Inventory Planning and Optimisation With Predictive Analytics: Insights into how predictive analytics can optimize inventory planning and management.
- Predictive Analytics Transforms Inventory Management in Retail | Retalon: An article discussing the impact of predictive analytics on retail inventory management.
Disclaimer
This is an AI-generated article with educative purposes and doesn’t intend to give advice or recommend its implementation. The goal is to inspire readers to research and delve deeper into the topics covered in the article.
- Signs You’re Understaffed - January 20, 2025
- The Cost of Silence: Communicating Negative Feedback - January 13, 2025
- Holacracy (Decentralized Management) - January 6, 2025