Deep learning can significantly enhance content generation by automating the creation process, personalizing content for users, and improving generated content quality. By leveraging neural networks, deep learning models can analyze vast amounts of data, learn patterns, and generate human-like text.
This technology can help businesses produce content at scale, maintain consistency, and reduce the time and resources needed for content creation.
What are the key benefits of using deep learning for content generation?
Deep learning offers several advantages for content generation:
- Automation: Deep learning models can automate repetitive tasks, such as writing product descriptions, generating reports, or creating social media posts. This frees up human resources for more strategic activities.
- Personalization: By analyzing user data, deep learning can create personalized content that resonates with individual users, improving engagement and user experience.
- Consistency: Deep learning ensures consistent tone, style, and quality across all generated content, maintaining brand voice and reducing the risk of errors.
- Scalability: Businesses can scale their content production without a proportional cost increase, making it easier to meet growing content demands.
How does deep learning improve the quality of generated content?
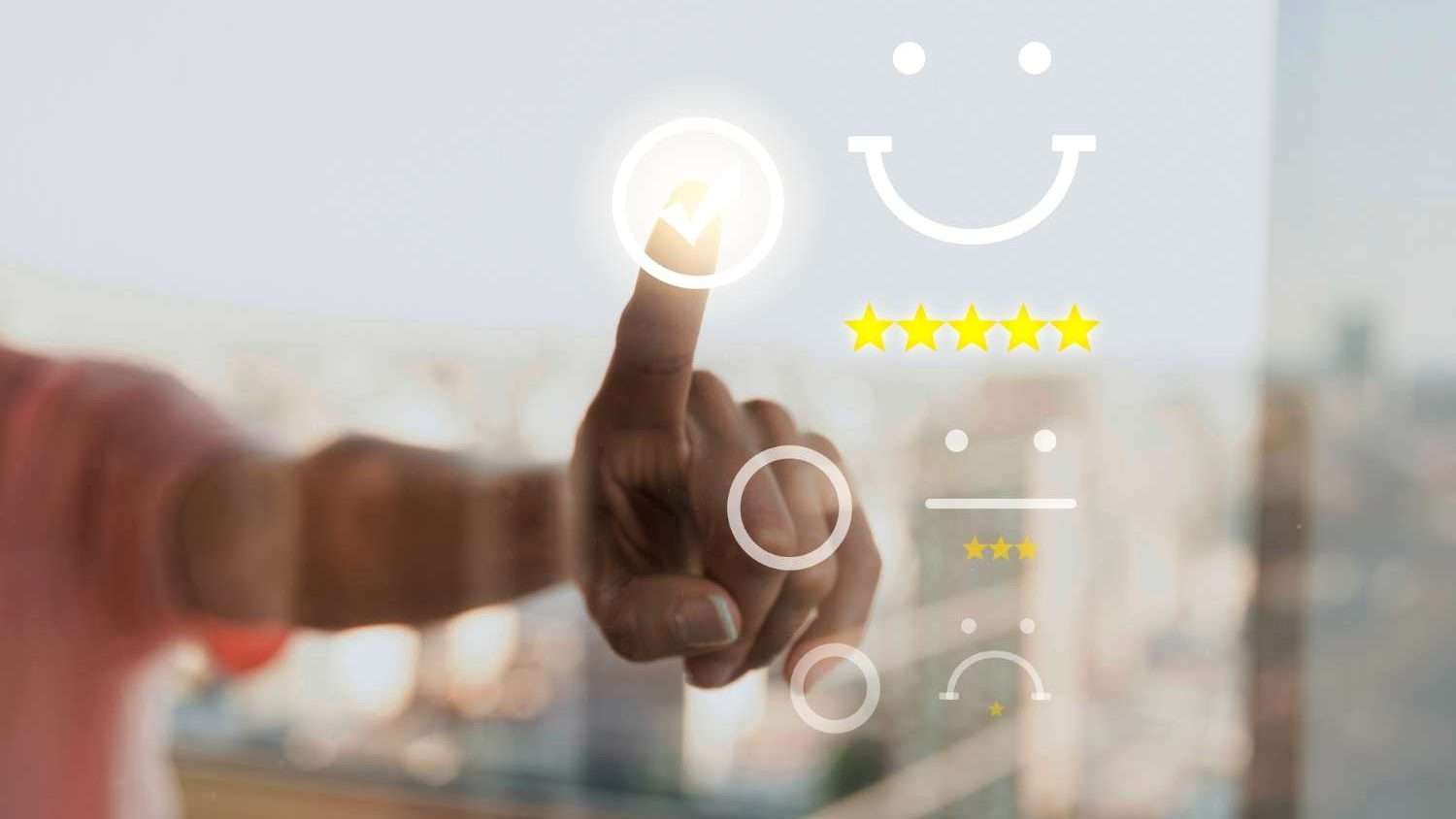
Deep learning improves the quality of generated content through several key mechanisms. One significant aspect is contextual understanding, where models like GPT-3 comprehend the context of a given topic and generate text that is coherent and relevant, thereby reducing the need for extensive editing and ensuring the content is logical and appropriate.
Another important factor is language proficiency. These models are trained on diverse datasets, which enables them to produce text that is grammatically correct and stylistically suitable for various contexts.
In addition, deep learning enhances creativity in content generation. Advanced models can emulate human writing styles and integrate unique ideas, resulting in creative and engaging content. Furthermore, the continuous learning process from user feedback allows these models to improve over time. This ongoing refinement helps in enhancing their ability to generate high-quality content consistently.
What are some practical applications of deep learning in content generation?
Deep learning can be applied in various content generation scenarios:
- Blog Writing: AI can draft blog posts based on given topics, keywords, and outlines, saving time for content creators.
- Social Media: Automated generation of social media posts, captions, and hashtags tailored to specific platforms and audiences.
- Product Descriptions: E-commerce platforms can use AI to create detailed and engaging product descriptions at scale.
- News Articles: AI can generate news summaries, reports, and articles, providing timely updates on various topics.
What are the challenges of using deep learning for content generation?
Despite its benefits, using deep learning for content generation faces several challenges. One major issue is quality control, as it can be difficult to ensure that the generated content meets quality standards and aligns with brand guidelines.
Additionally, bias and ethics are significant concerns because AI models can inadvertently produce biased or inappropriate content, leading to ethical dilemmas.
Another challenge is data dependency. Deep learning models require large datasets for effective training, but such data may not always be available or accessible. Finally, even though AI can automate content generation, human oversight remains essential to review and refine the output to ensure its accuracy and appropriateness.
For more insights on deep learning, check out our pillar article: How Does Deep Learning Work? Insights from Google’s AI Experts.
- Quantum Computing for Market Volatility Prediction - October 30, 2024
- Blockchain for Asset Ownership - October 23, 2024
- Blockchain-Enabled IoT Device Authentication - October 16, 2024