In the rapidly evolving field of artificial intelligence, the integration of human judgment in AI decision-making is becoming increasingly crucial.
This article explores the importance of human judgment in AI, the challenges faced in the industry, and potential solutions to enhance AI decision-making processes.
Understanding Human Judgment in AI
Key Points
- Human judgment is essential for contextual decision-making in AI.
- AI models often fail to replicate nuanced human decisions.
- Training data quality significantly impacts AI judgment accuracy.
- Combining human feedback with AI can improve decision outcomes.
- Transparency in data collection and labeling is critical.
Definition and Importance
Human judgment in AI refers to the integration of human insights and contextual understanding into AI decision-making processes. While AI excels at processing vast amounts of data and making predictions, it often lacks the nuanced understanding that humans bring to complex decisions. This human element is crucial for ensuring that AI systems make decisions that align with ethical standards and societal norms.
For instance, in legal or medical contexts, human judgment is indispensable. AI can assist by providing data-driven insights, but the final decision often requires a human touch to consider factors that AI might overlook. This combination of AI and human judgment can lead to more balanced and fair outcomes.
Moreover, human judgment helps in addressing biases that may be present in AI models. By incorporating human feedback, AI systems can be fine-tuned to better reflect real-world complexities and ethical considerations.
Challenges in Replicating Human Judgment
One of the significant challenges in integrating human judgment into AI is the difficulty in replicating the nuanced decisions that humans make. AI models are typically trained on large datasets, but these datasets may not capture the full spectrum of human decision-making. As a result, AI systems can sometimes make decisions that are harsher or more lenient than those a human would make.
For example, a study by MIT found that AI models trained to mimic human decision-making often suggest harsher judgments than humans would. This discrepancy arises because the data used to train these models may not accurately reflect the context in which human decisions are made. This highlights the importance of using high-quality, contextually relevant data for training AI models.
Additionally, the way data is labeled can impact the accuracy of AI judgments. Descriptive labels, which focus on factual features, may not be sufficient for training models that need to make normative judgments, such as determining whether a rule has been violated. This further underscores the need for careful consideration of data quality and labeling practices.
Combining AI and Human Judgment
To address these challenges, researchers and practitioners are exploring ways to combine AI with human judgment effectively. One approach is to use human feedback to fine-tune AI models. By incorporating human insights into the training process, AI systems can be better aligned with human values and ethical standards.
For instance, OpenAI has developed methods to teach AI human judgment using simple forms of human feedback through chat interactions. This allows humans to guide AI systems in understanding which outcomes are preferable in different contexts. Such approaches can help bridge the gap between AI predictions and human judgment, leading to more balanced and fair decision-making processes.
Another strategy is to improve transparency in data collection and labeling. By ensuring that data used to train AI models is gathered and labeled in a way that reflects the context of human decision-making, the accuracy and fairness of AI judgments can be enhanced. This requires collaboration between AI researchers, data scientists, and domain experts to develop robust data collection and labeling practices.
Challenges in the Industry
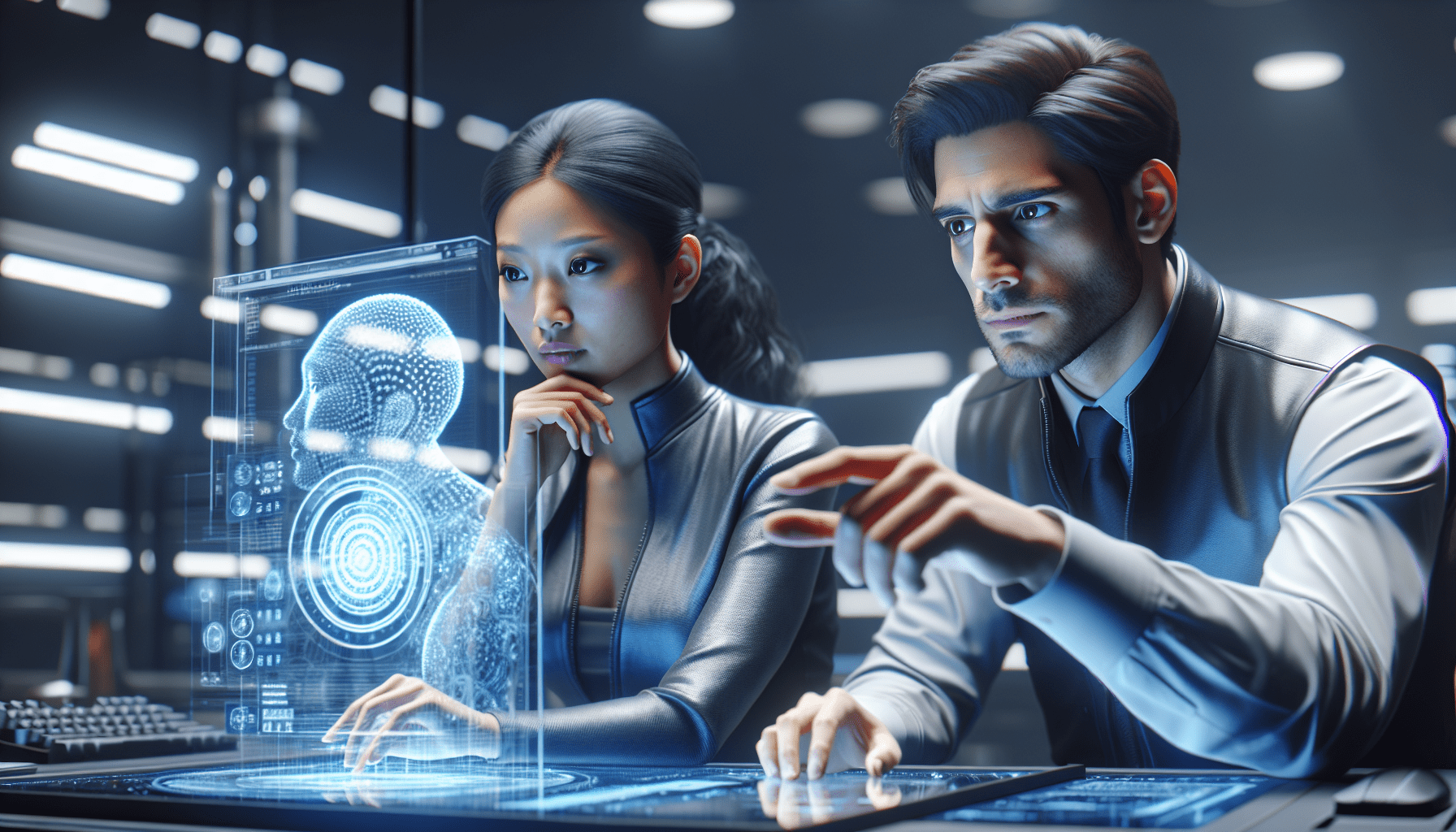
Bias in AI Decision-Making
One of the most pressing challenges in the industry is the presence of bias in AI decision-making. AI models are often trained on historical data, which may contain biases that reflect societal inequalities. When these biases are not addressed, AI systems can perpetuate and even amplify them, leading to unfair outcomes.
For example, in the context of hiring, AI systems trained on biased data may favor certain demographic groups over others. This can result in discriminatory hiring practices and limit opportunities for underrepresented groups. Addressing bias in AI decision-making is crucial for ensuring fairness and equity in AI applications.
Data Quality and Labeling
Another significant challenge is the quality and labeling of data used to train AI models. As mentioned earlier, the way data is labeled can impact the accuracy of AI judgments. Descriptive labels may not capture the full context of human decision-making, leading to discrepancies between AI and human judgments.
Ensuring that data is accurately labeled and reflects the context in which decisions are made is essential for improving the performance of AI models. This requires collaboration between data scientists, domain experts, and human labelers to develop robust data labeling practices.
Transparency and Accountability
Transparency and accountability are critical issues in the industry. AI systems often operate as “black boxes,” making it difficult to understand how decisions are made. This lack of transparency can lead to mistrust and skepticism among users and stakeholders.
To address this, there is a growing emphasis on developing explainable AI (XAI) systems that provide insights into how decisions are made. By making AI decision-making processes more transparent, stakeholders can better understand and trust the outcomes. Additionally, establishing accountability mechanisms ensures that AI systems are used responsibly and ethically.
Steps to Enhance AI Decision-Making
Step 1: Incorporate Human Feedback
One of the most effective ways to enhance AI decision-making is to incorporate human feedback into the training process. By involving humans in the loop, AI systems can be fine-tuned to better reflect human values and ethical standards. This can be achieved through methods such as human-in-the-loop (HITL) training, where humans provide feedback on AI predictions and decisions.
For example, in the context of content moderation, human moderators can review AI-generated decisions and provide feedback on whether they align with community guidelines. This feedback can then be used to improve the accuracy and fairness of AI models, ensuring that they make decisions that are consistent with human judgment.
Step 2: Improve Data Quality and Labeling
Improving the quality and labeling of data used to train AI models is another crucial step. This involves ensuring that data is accurately labeled and reflects the context in which decisions are made. By using normative labels that capture the context of human decision-making, AI models can be trained to make more accurate and fair judgments.
Collaboration between data scientists, domain experts, and human labelers is essential for developing robust data labeling practices. Additionally, transparency in data collection and labeling processes can help build trust and ensure that AI systems are used responsibly.
Step 3: Develop Explainable AI Systems
Developing explainable AI (XAI) systems is critical for enhancing transparency and accountability in AI decision-making. XAI systems provide insights into how decisions are made, making it easier for users and stakeholders to understand and trust the outcomes.
For instance, in the context of healthcare, XAI systems can provide explanations for AI-generated diagnoses and treatment recommendations. This allows healthcare professionals to understand the reasoning behind AI decisions and make informed choices. By making AI decision-making processes more transparent, XAI systems can help build trust and ensure that AI is used ethically and responsibly.
FAQs
Why is human judgment important in AI decision-making?
Human judgment is important in AI decision-making because it provides contextual understanding and ethical considerations that AI systems may lack. While AI excels at processing data and making predictions, it often requires human insights to make balanced and fair decisions.
How can bias in AI decision-making be addressed?
Bias in AI decision-making can be addressed by using high-quality, contextually relevant data and incorporating human feedback into the training process. Additionally, developing explainable AI systems can help identify and mitigate biases in AI models.
What is the role of data quality in AI decision-making?
Data quality plays a crucial role in AI decision-making as it directly impacts the accuracy and fairness of AI models. Ensuring that data is accurately labeled and reflects the context of human decision-making is essential for training effective AI systems.
What are explainable AI systems?
Explainable AI (XAI) systems provide insights into how AI decisions are made, making the decision-making process more transparent and understandable. XAI systems help build trust and ensure that AI is used responsibly and ethically.
Future of Human Judgment in AI
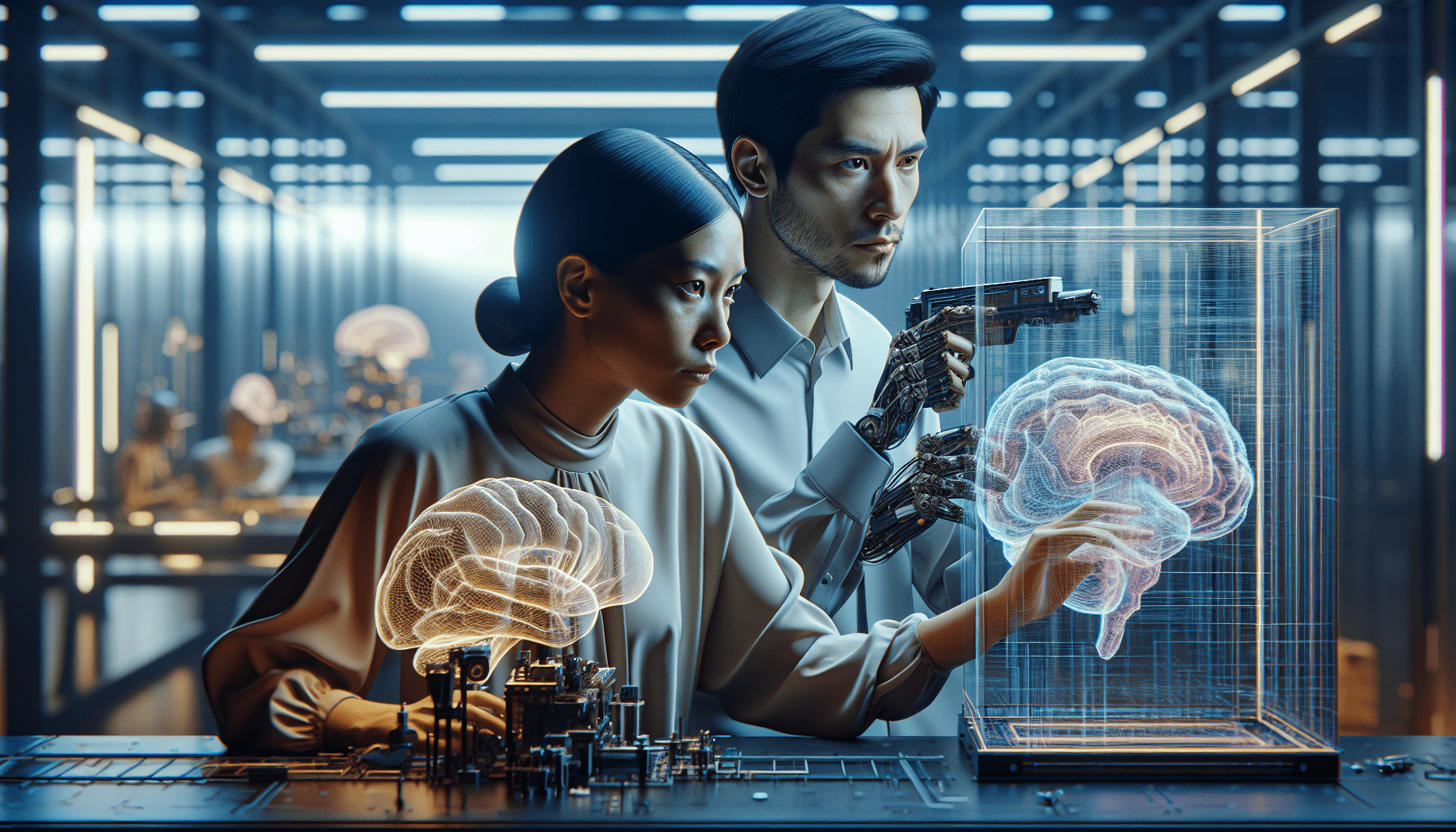
The future of human judgment in AI decision-making is poised to evolve significantly. Here are five robust predictions backed by real trend data:
- Increased Integration of Human Feedback: AI systems will increasingly incorporate human feedback to improve decision accuracy and fairness.
- Advancements in Explainable AI: The development of explainable AI systems will enhance transparency and accountability in AI decision-making.
- Improved Data Labeling Practices: Collaboration between data scientists, domain experts, and human labelers will lead to more accurate and contextually relevant data labeling.
- Ethical AI Frameworks: The establishment of ethical AI frameworks will guide the responsible and fair use of AI in various applications.
- Enhanced AI-Human Collaboration: The synergy between AI and human judgment will lead to more balanced and effective decision-making processes.
More Information
- How Large Language Models Reflect Human Judgment – An article exploring how large language models incorporate human judgment through feedback.
- Study: AI models fail to reproduce human judgements about rule violations | MIT News – A study highlighting the challenges AI models face in replicating human judgments.
- Human Judgment and AI Pricing – American Economic Association – A paper examining the role of human judgment in AI pricing decisions.
- Prediction and Judgment: Why Artificial Intelligence Needs Human Judgment – An article discussing the importance of human judgment in AI predictions.
Disclaimer
This is an AI-generated article with educative purposes and doesn’t intend to give advice or recommend its implementation. The goal is to inspire readers to research and delve deeper into the topics covered in the article.
- Burnout in Remote Teams: How It’s Draining Your Profits - January 27, 2025
- Signs You’re Understaffed - January 20, 2025
- The Cost of Silence: Communicating Negative Feedback - January 13, 2025